A multi-feature health factor fusion method based on sdrsn
A technology of health factors and fusion methods, applied in neural learning methods, testing of mechanical components, testing of machine/structural components, etc., to reduce the influence of empirical factors, remove redundant information, and avoid overfitting.
- Summary
- Abstract
- Description
- Claims
- Application Information
AI Technical Summary
Problems solved by technology
Method used
Image
Examples
specific Embodiment approach 1
[0051] Specific implementation mode 1: refer to figure 1 and figure 2 Specifically describe this embodiment, a multi-feature health factor fusion method based on SDRSN described in this embodiment is characterized by comprising:
[0052] Step 1: Collect the original vibration signal of the rotating machinery;
[0053] Step 2: perform smoothing and denoising preprocessing on the original vibration signal of the rotating machinery, and then extract the time domain, frequency domain and time-frequency domain features of the preprocessed original vibration signal of the rotating machinery, and construct the original feature set, Then normalize the signal in the original feature set;
[0054] Step 3: Use the normalized original feature set to filter and construct a sensitive feature set;
[0055] Step 4: Input the sensitive feature set into the SDRSN model for feature fusion training, input the data of the test set into the trained model, and obtain the health factor representi...
specific Embodiment approach 2
[0065] Embodiment 2: This embodiment is a further description of Embodiment 1. The difference between this embodiment and Embodiment 1 is that the final output of the model is expressed as:
[0066] x l+1 =x l +F(x l ,W l )
[0067] where x l Represents the output feature A, F(x l ,W l ) represents the output feature B.
specific Embodiment approach 3
[0068] Embodiment 3: This embodiment is a further description of Embodiment 2. The difference between this embodiment and Embodiment 2 is that the output feature of the convolutional layer is expressed as:
[0069] y 1 =∑x*k+b
[0070] where x is the input feature, k is the convolution kernel, and b is the bias.
PUM
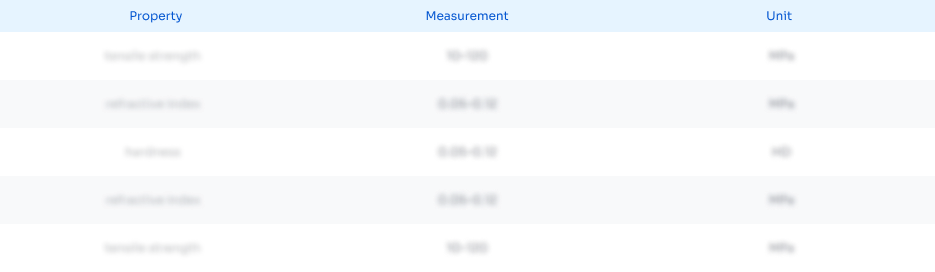
Abstract
Description
Claims
Application Information
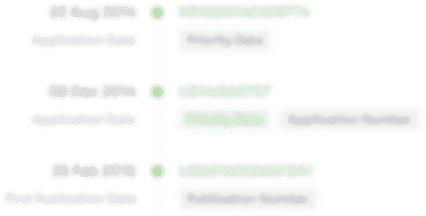
- R&D
- Intellectual Property
- Life Sciences
- Materials
- Tech Scout
- Unparalleled Data Quality
- Higher Quality Content
- 60% Fewer Hallucinations
Browse by: Latest US Patents, China's latest patents, Technical Efficacy Thesaurus, Application Domain, Technology Topic, Popular Technical Reports.
© 2025 PatSnap. All rights reserved.Legal|Privacy policy|Modern Slavery Act Transparency Statement|Sitemap|About US| Contact US: help@patsnap.com