Classification model establishment method based on dynamic joint distribution alignment and application thereof
A technology of joint distribution and classification model, applied in the field of domain adaptation, can solve problems such as lack of training data, and achieve the effect of reducing the number of modules, reducing resource and time consumption, and reducing resources and time
- Summary
- Abstract
- Description
- Claims
- Application Information
AI Technical Summary
Problems solved by technology
Method used
Image
Examples
Embodiment 1
[0055] A classification model building method based on dynamic joint distribution alignment, such as figure 1 shown, including the following steps:
[0056] (S1) Assign weights to the samples in the source domain dataset with category labels and the target domain dataset without category labels, so that the category distribution of the two datasets after weighting is the same; the feature spaces of the two datasets and The label space is the same, but the marginal and conditional distributions are different;
[0057] In this embodiment, the target domain data set belongs to the image classification task;
[0058] respectively with and Denotes the source domain dataset with category labels and the target domain dataset without category labels, where n s and n t Respectively represent the source domain dataset D s and the target domain dataset D t The number of samples in , (x i ,y i ) represents the source domain dataset D s samples in x i Indicates the image data ...
Embodiment 2
[0098] An image classification method comprising:
[0099] Input the image data to be classified in the image classification task into the classification model established by the classification model establishment method based on dynamic joint distribution alignment provided in the above-mentioned embodiment 1, so as to output the image classification result from the classification model.
[0100] Through the classification model building method based on dynamic joint distribution alignment provided by the above-mentioned embodiment 1, a classification model with good classification performance can be established in the absence of training data for image classification tasks. Therefore, based on the classification model, the present invention The provided image classification method can accurately complete image classification.
Embodiment 3
[0102] A method for establishing a classification model based on dynamic joint distribution alignment. This embodiment is similar to the above-mentioned Embodiment 1, except that in this embodiment, the target classification task is a text classification task.
PUM
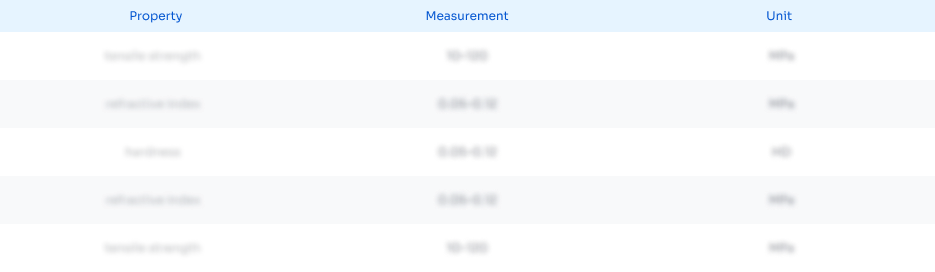
Abstract
Description
Claims
Application Information
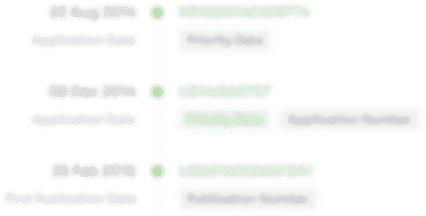
- R&D Engineer
- R&D Manager
- IP Professional
- Industry Leading Data Capabilities
- Powerful AI technology
- Patent DNA Extraction
Browse by: Latest US Patents, China's latest patents, Technical Efficacy Thesaurus, Application Domain, Technology Topic, Popular Technical Reports.
© 2024 PatSnap. All rights reserved.Legal|Privacy policy|Modern Slavery Act Transparency Statement|Sitemap|About US| Contact US: help@patsnap.com