Task scheduling method, device and equipment and computer storage medium
A task scheduling and task technology, applied in the field of deep learning technology, can solve problems such as unreasonable operator task scheduling and insufficient storage resources, and achieve the effect of alleviating insufficient storage resources and reasonable task scheduling.
- Summary
- Abstract
- Description
- Claims
- Application Information
AI Technical Summary
Problems solved by technology
Method used
Image
Examples
Embodiment 1
[0027] figure 1 The flow chart of the main method provided by Embodiment 1 of the present disclosure, in many application scenarios, it is necessary to perform multi-thread parallel scheduling on the device for target tasks, so as to improve computing efficiency. The aforementioned devices may be server devices, computer devices with relatively strong computing capabilities, and the like. The present disclosure can be applied to the above-mentioned devices. Such as figure 1 As shown in , the method may include the following steps:
[0028] In 101, according to the hardware execution cost of each operator task in the target task obtained through pre-analysis, the operator tasks that can be executed concurrently are prioritized.
[0029] The target task can be any computationally intensive task. A typical target task is a training task or an application task within a deep learning framework, that is, a training task or an application task based on a deep learning model.
[...
Embodiment 2
[0038] figure 2 A detailed method flow chart provided for Embodiment 2 of the present disclosure, such as figure 2 As shown in , the method may include the following steps:
[0039] In 201, the hardware occupation information of each operator task in the target task is determined in advance.
[0040] The hardware occupancy information may include newly added hardware resource occupancy, resource recovery after execution, and hardware execution cost.
[0041] This step can be used but not limited to the following two methods:
[0042] The first method: in the compilation stage of the target task, according to the size of the specified input data and the dependencies between the operator tasks in the target task, determine the hardware occupation information of each operator task.
[0043] For example, after a user builds a deep learning model, the size of the input data and output data of each operator task can be deduced in the model compilation stage according to the siz...
Embodiment 3
[0074] image 3 A schematic structural diagram of a task scheduling device provided in Embodiment 3 of the present disclosure. The device may be an application located on the server side, or may also be a functional unit such as a plug-in or a software development kit (Software Development Kit, SDK) in the application located on the server side. Alternatively, it may also be located at a computer terminal, which is not particularly limited in this embodiment of the present invention. Such as image 3 As shown in , the apparatus 300 may include: a sorting unit 301 and a scheduling unit 302 , and may further include a first analyzing unit 303 , a second analyzing unit 304 and a determining unit 305 . The main functions of each component unit are as follows:
[0075] The sorting unit 301 is configured to prioritize the operator tasks that can be executed concurrently according to the hardware execution cost of each operator task in the target task obtained through pre-analysis....
PUM
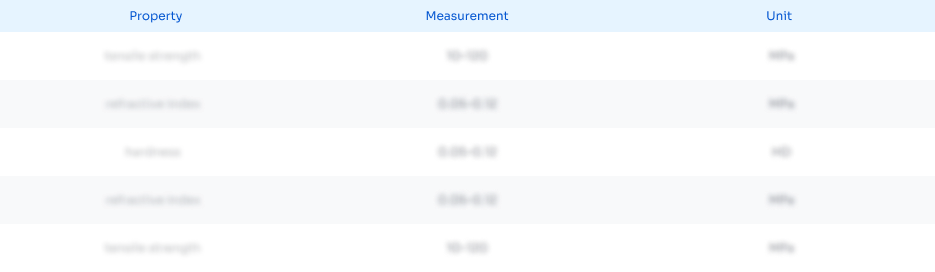
Abstract
Description
Claims
Application Information
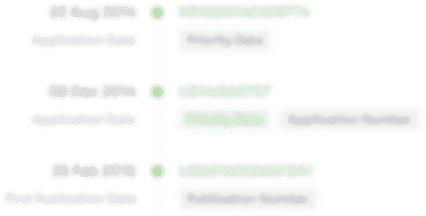
- R&D
- Intellectual Property
- Life Sciences
- Materials
- Tech Scout
- Unparalleled Data Quality
- Higher Quality Content
- 60% Fewer Hallucinations
Browse by: Latest US Patents, China's latest patents, Technical Efficacy Thesaurus, Application Domain, Technology Topic, Popular Technical Reports.
© 2025 PatSnap. All rights reserved.Legal|Privacy policy|Modern Slavery Act Transparency Statement|Sitemap|About US| Contact US: help@patsnap.com