Chemical fault diagnosis method based on unbalanced correction convolutional neural network
A convolutional neural network and fault diagnosis technology, which is applied in the chemical industry, can solve problems such as a small number of classifiers with few faults, reduced classification accuracy of classifiers, and inability of classifiers to learn category knowledge, so as to simplify the learning process and achieve remarkable robustness and reliability effects
- Summary
- Abstract
- Description
- Claims
- Application Information
AI Technical Summary
Problems solved by technology
Method used
Image
Examples
Embodiment 1
[0244] Example 1: Diagnostic model experiment of unbalanced fault data
[0245] In order to evaluate the performance of the present invention, six have a fault having a particular imbalance ratio during the training, wherein the failures 8 and 13 are a minority type of fault type. Such as Figure 6 As shown, you can find the advantages of diagnostic performance in a few fault mode. The present invention has a significant improvement in identifying a few faults compared to other methods. The performance of the present invention has nearly 6.7% and 2.9%, respectively, compared to prior art. Therefore, it is proved that the present invention has an advantage in generating a small number of fault samples. From Figure 6 It can be seen in the present invention better than the shallow model because it can effectively extract features from the original data and process unbalanced data in the complex chemistry. Since the deep architecture is employed, the present invention can effectively s...
Embodiment 2
[0251] Example 2: Diagnostic model experiment with increased type
[0252] Here, the incremental learning capability of the present invention is described herein for new samples and fault categories. The present invention can be adapted to be updated to a new fault. Here, the number of faults is gradually increased from 10 to 15. The top 10 faults experiment results Figure 9 (a) shown. It illustrates the new sample incremental learning ability. in Figure 9 In (a), the X axis represents the number of training samples per fault category, and the Y-axis represents the accuracy of the diagnostic model test sample. Each diagnostic model is initialized using 200 samples of each fault category. Then, for each step, 50 samples will be added to each fault category to test the incremental learning capabilities of the proposed method. In this case, SVM, BPNN, DBN, and CNN are fully trained based on the corresponding data set for comparison.
[0253] When there is a new fault category, the in...
PUM
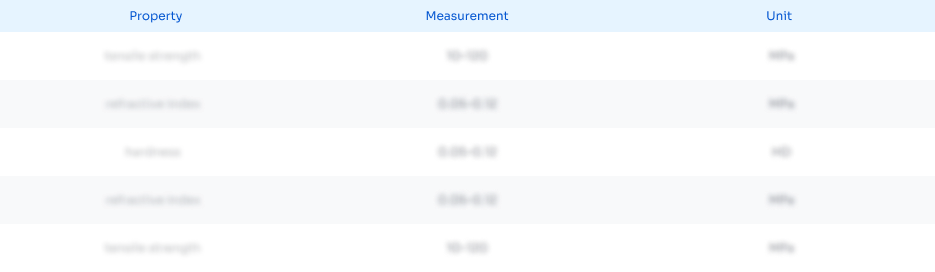
Abstract
Description
Claims
Application Information
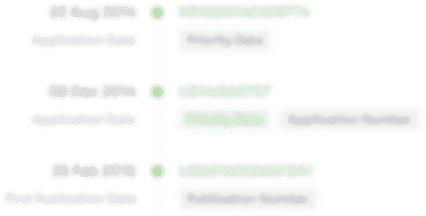
- R&D Engineer
- R&D Manager
- IP Professional
- Industry Leading Data Capabilities
- Powerful AI technology
- Patent DNA Extraction
Browse by: Latest US Patents, China's latest patents, Technical Efficacy Thesaurus, Application Domain, Technology Topic, Popular Technical Reports.
© 2024 PatSnap. All rights reserved.Legal|Privacy policy|Modern Slavery Act Transparency Statement|Sitemap|About US| Contact US: help@patsnap.com