HVAC control system based on multi-step prediction deep reinforcement learning algorithm
A multi-step prediction and reinforcement learning technology, applied in neural learning methods, prediction, system integration technology, etc., can solve problems such as difficult parameter adjustment, affecting modeling accuracy, and not considering the delay of HVAC systems
- Summary
- Abstract
- Description
- Claims
- Application Information
AI Technical Summary
Problems solved by technology
Method used
Image
Examples
Embodiment Construction
[0035] The present invention takes the collected real ambient temperature data as the experimental object, and trains and tests the HVAC control system based on the multi-step prediction depth reinforcement learning algorithm
[0036] The HVAC control system based on multi-step prediction deep reinforcement learning algorithm includes two stages: multi-step prediction of outdoor ambient temperature and real-time control of indoor temperature. The prediction stage of outdoor ambient temperature includes the following steps:
[0037] Step 1: According to the actual data points of the outdoor environment, select the outdoor ambient temperature X=[T 1 ,...,T i ] as the input of the model, h=[h i+1 ,...,h i+n ] as the true output of the model, the sampling interval is every 30 minutes.
[0038] Step 2: Preprocess the collected data, correct the abnormal data, convert the time series data into supervised sequence data, and divide the data into 2500 sets of training sets and 1000 ...
PUM
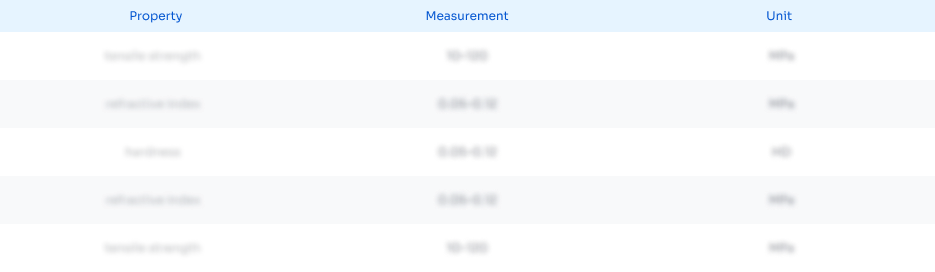
Abstract
Description
Claims
Application Information
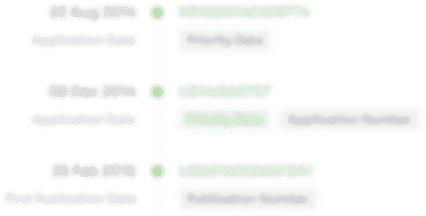
- R&D Engineer
- R&D Manager
- IP Professional
- Industry Leading Data Capabilities
- Powerful AI technology
- Patent DNA Extraction
Browse by: Latest US Patents, China's latest patents, Technical Efficacy Thesaurus, Application Domain, Technology Topic, Popular Technical Reports.
© 2024 PatSnap. All rights reserved.Legal|Privacy policy|Modern Slavery Act Transparency Statement|Sitemap|About US| Contact US: help@patsnap.com