Traffic prediction method based on graph transfer learning
A technology of traffic forecasting and transfer learning, which is applied to transfer specific time and space knowledge fields across domains, can solve problems such as low prediction accuracy, and achieve the effect of improving prediction accuracy
- Summary
- Abstract
- Description
- Claims
- Application Information
AI Technical Summary
Problems solved by technology
Method used
Image
Examples
Embodiment Construction
[0080] The present invention will be described in further detail below in conjunction with specific embodiments, but the present invention is not limited to specific embodiments.
[0081] A traffic migration prediction method based on graph convolutional neural network, including pre-training on data-rich domains to acquire knowledge and transfer knowledge to data-scarce domains and fine-tuning two parts:
[0082] 1. Train the network model on a data-rich domain to obtain spatial and temporal pattern information. The steps are as follows:
[0083] a) Assume that the number of model training rounds is M, there are K types of spatial patterns, Q types of temporal patterns, and the smoothing coefficients are γ and θ. We randomly choose E i K points in (i=1,2,...,N) are used as the initial center of the spatial pattern k∈{1,2,...,K}, choose R randomly i Q points in (i=1,2,...,N) are used as the initial center of the time pattern q∈{1,2,...,Q};
[0084] b) For e=1,2,...,Mdo:...
PUM
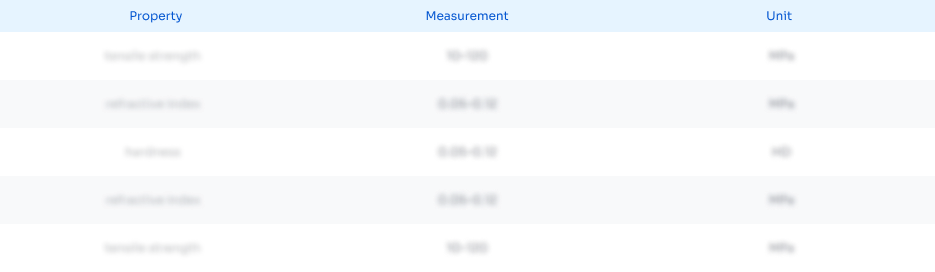
Abstract
Description
Claims
Application Information
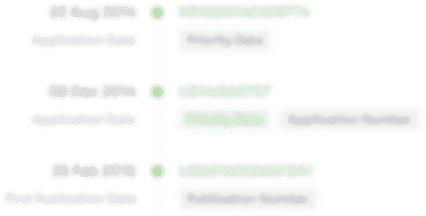
- R&D Engineer
- R&D Manager
- IP Professional
- Industry Leading Data Capabilities
- Powerful AI technology
- Patent DNA Extraction
Browse by: Latest US Patents, China's latest patents, Technical Efficacy Thesaurus, Application Domain, Technology Topic, Popular Technical Reports.
© 2024 PatSnap. All rights reserved.Legal|Privacy policy|Modern Slavery Act Transparency Statement|Sitemap|About US| Contact US: help@patsnap.com