Semi-supervised object pose estimation method combining generated data and unlabeled data
A technology for pose estimation and labeling data, applied in the field of robot vision, can solve the problems of insufficient accuracy and uneliminated differences in the field, and achieve the effect of improving flexibility and accuracy
- Summary
- Abstract
- Description
- Claims
- Application Information
AI Technical Summary
Problems solved by technology
Method used
Image
Examples
Embodiment Construction
[0032] The present invention will be described in detail below in conjunction with the accompanying drawings and specific embodiments. This embodiment is carried out on the premise of the technical solution of the present invention, and detailed implementation and specific operation process are given, but the protection scope of the present invention is not limited to the following embodiments.
[0033] This embodiment provides a semi-supervised object pose estimation method based on generated data and unlabeled real data. The schematic diagram of the method is as follows figure 1 As shown, it specifically includes the following steps:
[0034] S1, using the CAD model of the object to generate object point cloud data with pose tags;
[0035] S2. Obtain the unlabeled color image and depth image of the target object, input the color image to the trained instance segmentation network, obtain the instance segmentation result, and obtain the point cloud of the target object from t...
PUM
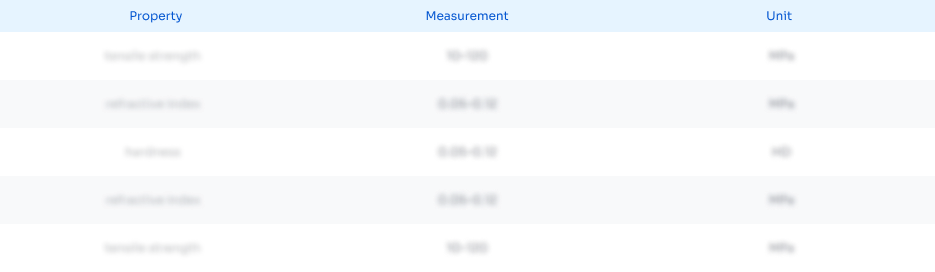
Abstract
Description
Claims
Application Information
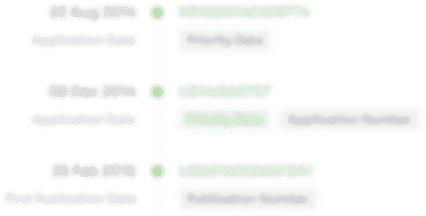
- R&D Engineer
- R&D Manager
- IP Professional
- Industry Leading Data Capabilities
- Powerful AI technology
- Patent DNA Extraction
Browse by: Latest US Patents, China's latest patents, Technical Efficacy Thesaurus, Application Domain, Technology Topic, Popular Technical Reports.
© 2024 PatSnap. All rights reserved.Legal|Privacy policy|Modern Slavery Act Transparency Statement|Sitemap|About US| Contact US: help@patsnap.com