Conversion method of high-precision low-delay pulse neural network
A technology of spiking neural network and conversion method, which is applied in neural learning methods, biological neural network models, neural architectures, etc., can solve problems such as insufficient network performance, and achieve the effects of fine-tuning training data, network performance promotion, and low latency.
- Summary
- Abstract
- Description
- Claims
- Application Information
AI Technical Summary
Problems solved by technology
Method used
Image
Examples
Embodiment
[0059] Such as figure 1 and figure 2 As shown, the conversion method of the high-precision low-latency spiking neural network includes the following steps:
[0060] S100. Construct an artificial neural network ANN with the same structure as the target pulse neural network SNN, and add a BN layer on the ANN network, configure the activation function as a ReLU activation function, and then use the training set data and the backpropagation algorithm to train the ANN network ;
[0061] S200. After the network training is completed, fold the BN layer into the weight and bias, and modify the average pooling layer in the ANN network structure to a convolutional layer with average weight;
[0062] S300. Copy the weight and deviation of the ANN network to the corresponding layer in the target SNN network, modify the ReLU activation function to an IF model, and set the simulation time;
[0063] S400. Select a batch of training set data, find the optimal threshold of the input layer...
PUM
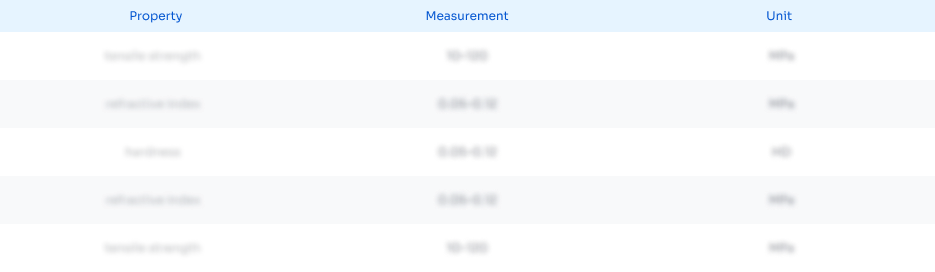
Abstract
Description
Claims
Application Information
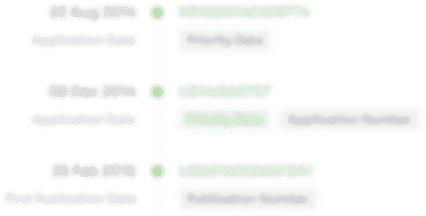
- R&D
- Intellectual Property
- Life Sciences
- Materials
- Tech Scout
- Unparalleled Data Quality
- Higher Quality Content
- 60% Fewer Hallucinations
Browse by: Latest US Patents, China's latest patents, Technical Efficacy Thesaurus, Application Domain, Technology Topic, Popular Technical Reports.
© 2025 PatSnap. All rights reserved.Legal|Privacy policy|Modern Slavery Act Transparency Statement|Sitemap|About US| Contact US: help@patsnap.com