Rolling bearing fault diagnosis method fusing attention mechanism and twin network structure
A network structure and rolling bearing technology, applied in the testing of machines/structural components, biological neural network models, neural learning methods, etc., can solve problems that are difficult to implement, a large number of fault sample data, etc., and achieve high scalability, accuracy and speed The effect of balance, powerful feature extraction and ability to process long time series
- Summary
- Abstract
- Description
- Claims
- Application Information
AI Technical Summary
Problems solved by technology
Method used
Image
Examples
Embodiment Construction
[0051] The present invention will be described in detail below in conjunction with the accompanying drawings and specific embodiments.
[0052] The present invention is a rolling bearing fault diagnosis method that integrates attention mechanism and twin network structure. figure 1 As shown, the special architecture of the Siamese network is mainly used to measure the similarity of two input samples, and the training of the model is completed by making the samples of the same type as close as possible and the samples of different types as far away as possible. This model can effectively solve the problem of insufficient model training due to the scarcity of fault sample data. Dynamic convolution dynamically aggregates multiple parallel convolution kernels according to attention, which greatly improves the size and capacity of the model while ensuring computational efficiency, and makes the network achieve a balance between accuracy improvement and running time. The effective ...
PUM
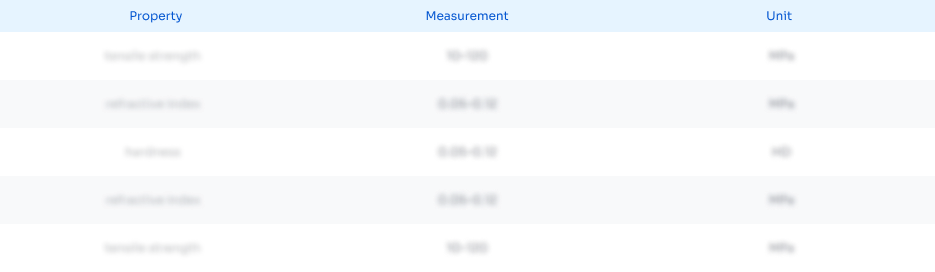
Abstract
Description
Claims
Application Information
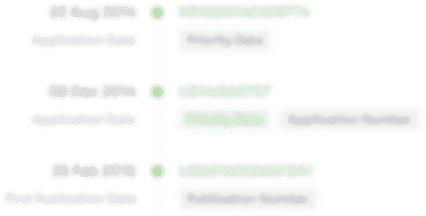
- R&D Engineer
- R&D Manager
- IP Professional
- Industry Leading Data Capabilities
- Powerful AI technology
- Patent DNA Extraction
Browse by: Latest US Patents, China's latest patents, Technical Efficacy Thesaurus, Application Domain, Technology Topic.
© 2024 PatSnap. All rights reserved.Legal|Privacy policy|Modern Slavery Act Transparency Statement|Sitemap