Multi-party collaborative model updating method, device and system for realizing privacy protection
A privacy protection and model update technology, applied in the computer field, can solve problems such as high communication overhead and privacy leakage
- Summary
- Abstract
- Description
- Claims
- Application Information
AI Technical Summary
Problems solved by technology
Method used
Image
Examples
Embodiment Construction
[0043] The solutions provided in this specification will be described below in conjunction with the accompanying drawings.
[0044] As mentioned earlier, traditional federated learning is achieved by sharing model parameters or gradients among various parties. Among them, the mainstream schemes are mainly divided into two types: the first, federated learning based on Central Differential Privacy (CDP); the second, federated learning based on Local Differential Privacy (LDP). The two methods will be described below in conjunction with the accompanying drawings.
[0045] figure 1 It is a schematic diagram of federated learning based on centralized differential privacy. figure 1 In , firstly, each participant uploads its own model gradients: Δw1, Δw2, ..., Δwn to a trusted third-party server (hereinafter referred to as the server). Afterwards, the server aggregates the model gradients uploaded by each participant: aggregate(Δw1+Δw2+…+Δwn), and adds noise to the aggregated mode...
PUM
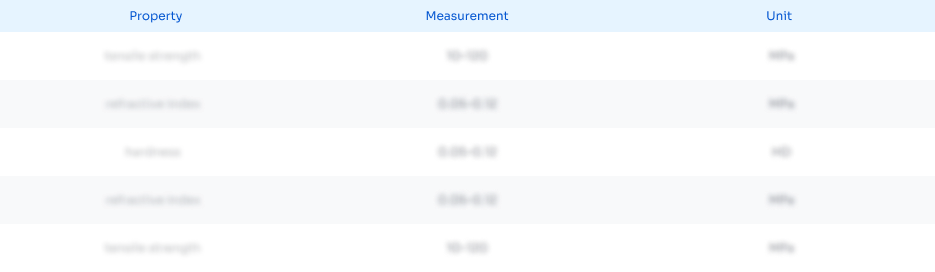
Abstract
Description
Claims
Application Information
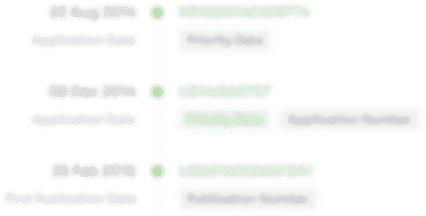
- R&D Engineer
- R&D Manager
- IP Professional
- Industry Leading Data Capabilities
- Powerful AI technology
- Patent DNA Extraction
Browse by: Latest US Patents, China's latest patents, Technical Efficacy Thesaurus, Application Domain, Technology Topic, Popular Technical Reports.
© 2024 PatSnap. All rights reserved.Legal|Privacy policy|Modern Slavery Act Transparency Statement|Sitemap|About US| Contact US: help@patsnap.com