Remote sensing image multi-label classification method based on adjacent matrix guide label embedding
An adjacency matrix and remote sensing image technology, applied in the field of image processing, can solve the problems of inability to associate multi-label and remote sensing image learning, lack of prior information of label feature vectors, affecting classification accuracy, etc., to eliminate difficult to characterize label dependencies, reduce Influence and increase the effect of mF1 value
- Summary
- Abstract
- Description
- Claims
- Application Information
AI Technical Summary
Problems solved by technology
Method used
Image
Examples
Embodiment Construction
[0040] The present invention will be further described in detail below in conjunction with the accompanying drawings and specific embodiments.
[0041] refer to figure 1 , the present invention includes the following steps.
[0042] Step 1) Obtain training sample set, test sample set, adjacency matrix and label vector matrix:
[0043] (1a) Obtain S pieces of optical remote sensing images containing C target categories X={X s |1≤s≤S}, each optical remote sensing image X s Contains at least one target category Each target category is included in P optical remote sensing images, where S≥100, C≥2, X s Indicates the sth optical remote sensing image, P≥2, , means X s contains the lth target category, , means X s does not contain the l-th target category. Wherein, S=2100, C=17.
[0044] (1b) For each optical remote sensing image X s Label the included targets to get a multi-label set L={L l |0≤l≤C-1}, and subtract the image mean value in the ImageNet dataset from each ...
PUM
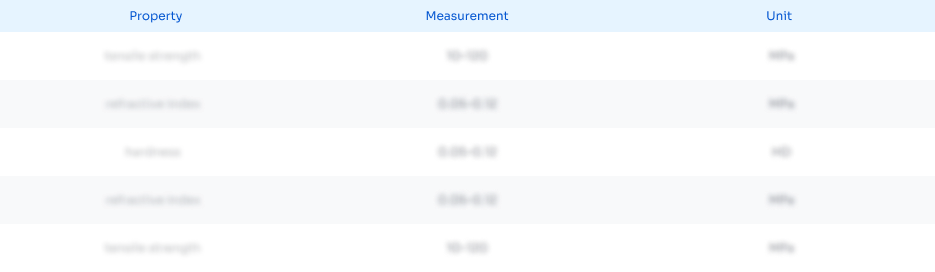
Abstract
Description
Claims
Application Information
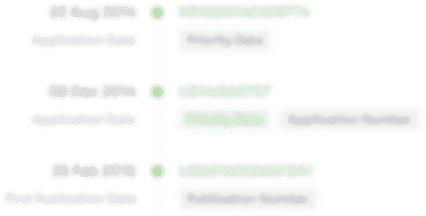
- R&D Engineer
- R&D Manager
- IP Professional
- Industry Leading Data Capabilities
- Powerful AI technology
- Patent DNA Extraction
Browse by: Latest US Patents, China's latest patents, Technical Efficacy Thesaurus, Application Domain, Technology Topic, Popular Technical Reports.
© 2024 PatSnap. All rights reserved.Legal|Privacy policy|Modern Slavery Act Transparency Statement|Sitemap|About US| Contact US: help@patsnap.com