Lightweight image super-resolution reconstruction method based on multi-dimensional knowledge distillation
A super-resolution reconstruction and super-resolution technology, which is applied in the field of lightweight image super-resolution reconstruction based on multi-dimensional knowledge distillation, can solve the problem of ignoring the multi-dimensional data set constraint solution space, consume resources, and cannot fully reflect Image degradation and other issues
- Summary
- Abstract
- Description
- Claims
- Application Information
AI Technical Summary
Problems solved by technology
Method used
Image
Examples
Embodiment 1
[0063] like figure 1 As shown, this embodiment provides a lightweight image super-resolution reconstruction method based on multi-dimensional knowledge distillation, including the following steps:
[0064] S1: Teacher network preprocessing: For the existing network, if there is a trained network model provided, it will be loaded directly, while for the undisclosed network model, it will be pre-trained first, and the training process of the teacher network refers to its original Training method, obtain and save the trained network model, and then load it.
[0065] In this embodiment, the teacher network used is the residual feature distillation network RFDN and the edge-enhanced super-resolution network Edge-SRN.
[0066] S2: Dataset preprocessing: Data enhancement is performed on the low-resolution images in the dataset, format conversion and grayscale processing are performed, and random cropping is performed. The processed low-resolution images are used for supervised netwo...
Embodiment 2
[0098] This embodiment provides a lightweight image super-resolution reconstruction system based on multi-dimensional knowledge distillation, including: a teacher network loading module, a data set preprocessing module, a student network construction module, a network model training module and a reconstruction module;
[0099] The teacher network loading module is used to preprocess and load the teacher network: for the untrained network, pre-train and obtain the model; for the trained and saved model, load the model. The selection of the teacher network in the multi-teacher network, the teacher network supervises the training of the student network for different dimensions.
[0100] In this embodiment, the multi-teacher network is composed of two kinds of teacher networks, namely: an index teacher network and a perception teacher network.
[0101] In this embodiment, the index teacher network is a trained residual feature distillation network (RFDN), which has the characteris...
Embodiment 3
[0123] This embodiment provides a storage medium, the storage medium can be a storage medium such as ROM, RAM, magnetic disk, optical disk, etc., and the storage medium stores one or more programs. A Lightweight Image Super-Resolution Reconstruction Method for Multidimensional Knowledge Distillation. .
PUM
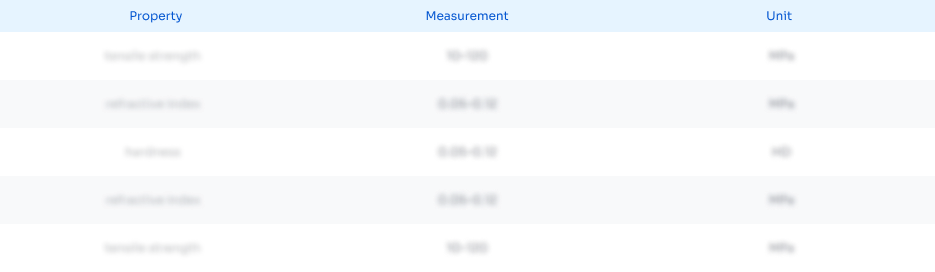
Abstract
Description
Claims
Application Information
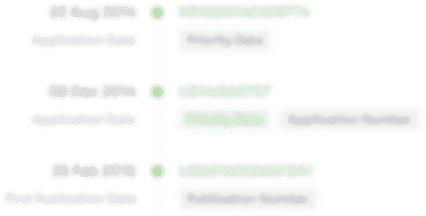
- R&D Engineer
- R&D Manager
- IP Professional
- Industry Leading Data Capabilities
- Powerful AI technology
- Patent DNA Extraction
Browse by: Latest US Patents, China's latest patents, Technical Efficacy Thesaurus, Application Domain, Technology Topic, Popular Technical Reports.
© 2024 PatSnap. All rights reserved.Legal|Privacy policy|Modern Slavery Act Transparency Statement|Sitemap|About US| Contact US: help@patsnap.com