Unprotected left-turn driving control method based on deep reinforcement learning
A technology of reinforcement learning and control methods, applied in neural learning methods, biological neural network models, neural architectures, etc., can solve problems such as limiting model training efficiency and error correction ability, and achieve the effect of improving interpretability
- Summary
- Abstract
- Description
- Claims
- Application Information
AI Technical Summary
Problems solved by technology
Method used
Image
Examples
Embodiment 1
[0042] Embodiment 1: as Figure 1 to Figure 5 As shown, the present invention provides an unprotected left-turn driving control method based on deep reinforcement learning. In order to create enough unprotected LTAP / OD events in one simulation, two identical closed road loop simulation scenarios were constructed ,Such as Figure 2a shown. It can be seen that after the two vehicles pass through the target intersection (frame selection position) of concern, they will come back through the loop to form a loop. Any number of unprotected LTAP / OD events can be obtained by setting an appropriate simulation run time. For the training process of deep reinforcement learning, each unprotected LTAP / OD event becomes an episode (Episode). Training and testing also need to use the scene of multiple straight vehicles (see Figure 2b ) and three candidate paths (see Figure 2c ).
[0043]Agents are expected to master human-like negotiation skills to handle complex unprotected LTAP / OD eve...
PUM
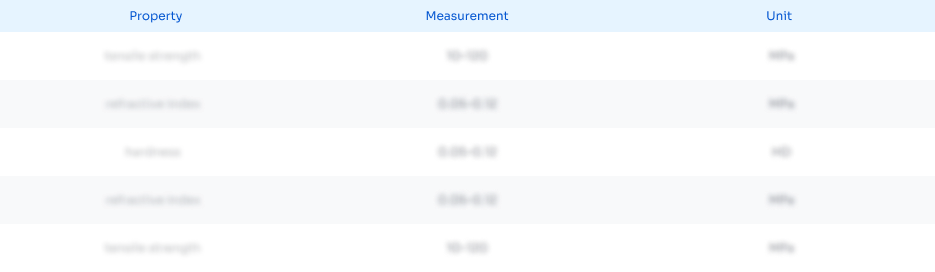
Abstract
Description
Claims
Application Information
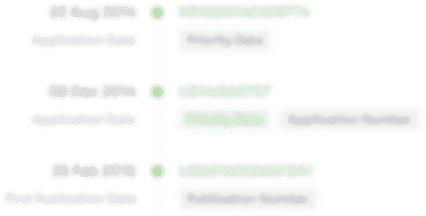
- R&D Engineer
- R&D Manager
- IP Professional
- Industry Leading Data Capabilities
- Powerful AI technology
- Patent DNA Extraction
Browse by: Latest US Patents, China's latest patents, Technical Efficacy Thesaurus, Application Domain, Technology Topic, Popular Technical Reports.
© 2024 PatSnap. All rights reserved.Legal|Privacy policy|Modern Slavery Act Transparency Statement|Sitemap|About US| Contact US: help@patsnap.com