Graph neural network recommendation method based on multi-aspect enhancement
A neural network and recommendation method technology, applied in the field of data mining, can solve problems such as ignoring user intentions and failing to capture fine-grained
- Summary
- Abstract
- Description
- Claims
- Application Information
AI Technical Summary
Problems solved by technology
Method used
Image
Examples
Embodiment Construction
[0069] In order to make the purpose, technical solution and advantages of the present invention more clear, the present invention will be further described in detail below in conjunction with the accompanying drawings and embodiments. It should be understood that the specific embodiments described here are only used to explain the present invention, not to limit the present invention.
[0070] The graph neural network recommendation method based on multi-aspect enhancement uses the BERT model to learn the user's aspect emotional characteristics, and constructs a heterogeneous aspect-aware graph. High-order neighbor aggregation is used to capture fine-grained preference features; then a routing-based fusion module is used to adaptively fuse various features to obtain a unified representation of users and items, thereby improving the recommendation performance and interpretability of the recommendation system.
[0071] Such as figure 1 As shown, the present invention discloses ...
PUM
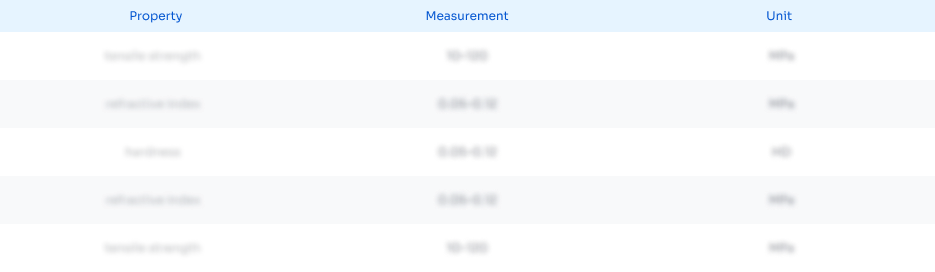
Abstract
Description
Claims
Application Information
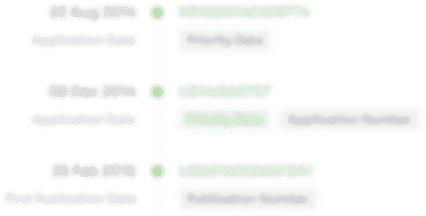
- R&D Engineer
- R&D Manager
- IP Professional
- Industry Leading Data Capabilities
- Powerful AI technology
- Patent DNA Extraction
Browse by: Latest US Patents, China's latest patents, Technical Efficacy Thesaurus, Application Domain, Technology Topic, Popular Technical Reports.
© 2024 PatSnap. All rights reserved.Legal|Privacy policy|Modern Slavery Act Transparency Statement|Sitemap|About US| Contact US: help@patsnap.com