Imperceptible watermark attack method based on residual learning, storage medium and electronic device
A watermarking attack and residual technology, applied in image watermarking, image data processing, instruments, etc., can solve problems such as interference and immature attack system
- Summary
- Abstract
- Description
- Claims
- Application Information
AI Technical Summary
Problems solved by technology
Method used
Image
Examples
Embodiment approach
[0080] S2. Based on the constructed watermark attack model, design a loss function; including selecting an appropriate number of feature extraction blocks according to the embedding area of the watermark information to extract a feature map containing a large amount of watermark information; introducing a residual learning mechanism, by learning the watermark The difference between the image and the unwatermarked image is used to improve the convergence speed and learning ability of the watermark attack model. During specific implementation, as a preferred embodiment of the present invention, it specifically includes the following steps:
[0081] Step 1.1, the watermark attack model extracts the low-frequency feature image representing the watermark information through the feature extraction block, and in order to destroy the watermark information in the watermarked image, the watermarked image is subtracted from the low-frequency feature image to obtain a residual image; nam...
Embodiment 1
[0118] In order to verify the effectiveness of the inventive method, a simulation experiment was carried out, and the following experimental results were given, and the experimental results were analyzed simultaneously, specifically as follows:
[0119] 1. Embedding area for watermark information:
[0120] The method of color image robust watermarking based on quaternion exponent moments embeds watermark information into the specific area of the original image without watermark, which determines the structural design of the watermark attack model. If the watermark information is embedded in the high-frequency region of the original image without watermark, then the feature extraction module of the watermark attack model is mainly used to extract the high-frequency information of the watermarked image, and the residual image (including the watermarked image and the extracted feature image The watermark information that is poorly done) will be destroyed to the greatest extent;...
Embodiment 2
[0126] 2. For the depth of network architecture
[0127] In order to verify the impact of the depth of the network architecture on the attack capability of the model, the feature extraction blocks of this embodiment are respectively selected as 7, 11, 15 and 20, and 25 watermarked images are randomly selected from the test set to calculate the bit error of extracting the watermark information rate, the experimental results such as Figure 5 shown, from Figure 5 It can be seen from the experimental results that when the number of layers N of the feature extraction block is 2, the average bit error rate of watermark information extraction is 0.11716, indicating that the watermark attack model has a certain destructive ability; while the number of layers N of the feature extraction block When 7 is selected, the average bit error rate of watermark information extraction is as high as 0.13885. It can be concluded that as the depth of the model increases, the attack capability of ...
PUM
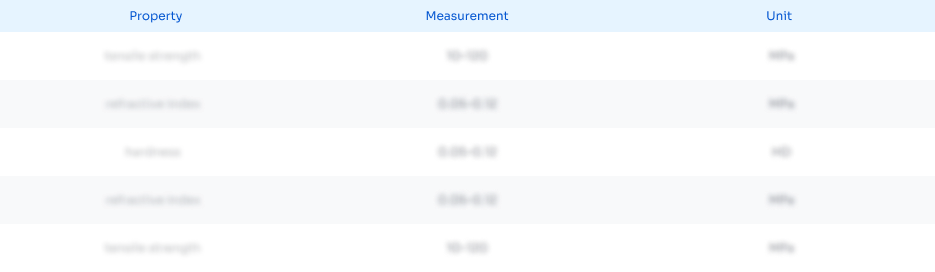
Abstract
Description
Claims
Application Information
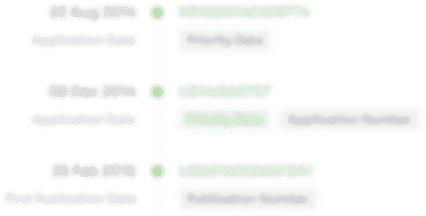
- R&D Engineer
- R&D Manager
- IP Professional
- Industry Leading Data Capabilities
- Powerful AI technology
- Patent DNA Extraction
Browse by: Latest US Patents, China's latest patents, Technical Efficacy Thesaurus, Application Domain, Technology Topic.
© 2024 PatSnap. All rights reserved.Legal|Privacy policy|Modern Slavery Act Transparency Statement|Sitemap