End-to-end speech recognition method based on constrained structured sparse attention mechanism, and storage medium
A speech recognition and attention technology, applied in speech recognition, speech analysis, instruments, etc., can solve problems such as interfering with the decoder recognition process
- Summary
- Abstract
- Description
- Claims
- Application Information
AI Technical Summary
Problems solved by technology
Method used
Image
Examples
specific Embodiment approach 1
[0053] This embodiment is an end-to-end speech recognition method based on a constraint-based structured sparse attention mechanism, such as figure 1 As shown, in the training phase, firstly, the original signal from the training set is sampled, quantized, frame-level feature extraction, high-level acoustic representation extraction, matching score calculation; then, through matching score sorting, matching score threshold calculation, attention Score normalization and glance vector generation are used to obtain glance vectors at each decoding moment; finally, the decoder is used for recognition and used to train the recognizer to obtain a speech recognition model. In the test phase, first, sample, quantize, and extract frame-level features for each original speech signal in the test set; then, use the trained speech recognition model to perform high-level acoustic representation extraction and matching score calculation on the feature matrix; next , through matching score sor...
Embodiment
[0077] In order to verify the effect of the present invention, the LibriSpeech data set is processed using the end-to-end speech recognition method based on the restricted structured sparse attention mechanism described in Embodiment 1, and with related methods (traditional softmax attention mechanism The processing method) is compared with the processing effect of the LibriSpeech data set, such as figure 2 The displayed accuracy rate comparison histogram is shown, where CER and WER represent the word error rate and word error rate respectively, and dev and test represent the processing accuracy rates of the development process and the test process, respectively. By comparing the accuracy of the method proposed in the present invention and the end-to-end speech recognition method based on the Softmax transformation function, it can be verified that the limited structured sparse attention mechanism can effectively reduce the word error rate and word error rate, and the effect i...
specific Embodiment approach 2
[0079] This embodiment is a storage medium, and at least one instruction is stored in the storage medium, and the at least one instruction is loaded and executed by a processor to implement an end-to-end speech recognition method based on a constraint-based structured sparse attention mechanism.
PUM
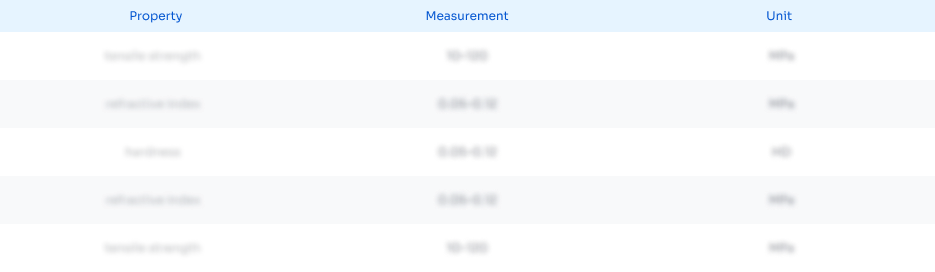
Abstract
Description
Claims
Application Information
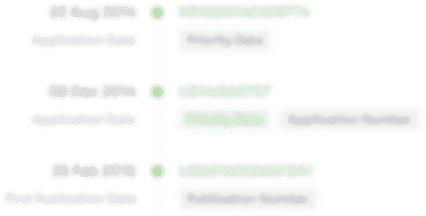
- R&D Engineer
- R&D Manager
- IP Professional
- Industry Leading Data Capabilities
- Powerful AI technology
- Patent DNA Extraction
Browse by: Latest US Patents, China's latest patents, Technical Efficacy Thesaurus, Application Domain, Technology Topic, Popular Technical Reports.
© 2024 PatSnap. All rights reserved.Legal|Privacy policy|Modern Slavery Act Transparency Statement|Sitemap|About US| Contact US: help@patsnap.com