Hyperspectral image semi-supervised classification method based on small sample learning
A hyperspectral image and classification method technology, applied in the field of hyperspectral image classification, to achieve the effect of improving classification accuracy, reasonable prototype calculation process, and alleviating over-fitting problems
- Summary
- Abstract
- Description
- Claims
- Application Information
AI Technical Summary
Problems solved by technology
Method used
Image
Examples
Embodiment Construction
[0031] Embodiments and effects of the present invention will be further described in detail below in conjunction with the accompanying drawings.
[0032] refer to figure 1 , the implementation steps of the present invention include as follows:
[0033] Step 1. Acquire five publicly available hyperspectral datasets.
[0034] Select five data sets of Indian Pines, KSC, Salinas, Pavia University and Botswana from the hyperspectral database, read them separately, and get the three-dimensional matrix data domain of each data set is m×n×h, and its label domain is two-dimensional Matrix m×n, where h represents the spectral dimension of the hyperspectral image, and (m,n) represents the position of a pixel on a certain spectrum.
[0035] In step 2, data preprocessing is performed on the three-dimensional matrix data domains in the five acquired data sets to eliminate the influence of noise and redundant information.
[0036] (2.1) Transform the three-dimensional matrix data domain m...
PUM
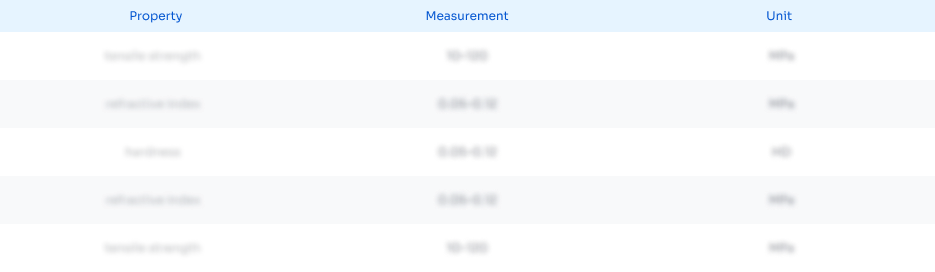
Abstract
Description
Claims
Application Information
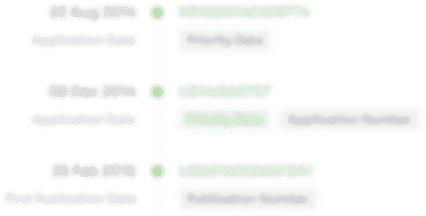
- Generate Ideas
- Intellectual Property
- Life Sciences
- Materials
- Tech Scout
- Unparalleled Data Quality
- Higher Quality Content
- 60% Fewer Hallucinations
Browse by: Latest US Patents, China's latest patents, Technical Efficacy Thesaurus, Application Domain, Technology Topic, Popular Technical Reports.
© 2025 PatSnap. All rights reserved.Legal|Privacy policy|Modern Slavery Act Transparency Statement|Sitemap|About US| Contact US: help@patsnap.com