Video Description Method for Semantic Reconstruction Based on Temporal Gaussian Mixture Atrous Convolution
A Gaussian mixture and video description technology, applied in the computer field, can solve problems such as gradient explosion, ignoring semantic differences at the sentence level, difficulty in effectively capturing long-term video sequence information, etc., to achieve the effect of narrowing semantic differences and reducing the amount of training parameters
- Summary
- Abstract
- Description
- Claims
- Application Information
AI Technical Summary
Problems solved by technology
Method used
Image
Examples
Embodiment Construction
[0039] The present invention will be further described below in conjunction with accompanying drawing.
[0040] Such as figure 1 , a semantic reconstruction video description method based on temporal Gaussian mixture atrous convolution. This method first uniformly samples the original video, uses convolutional neural network to extract appearance features and action features, and splicing according to the feature dimension to obtain video features; The Gaussian mixture hole convolution encoder obtains the temporal Gaussian video features; then the temporal Gaussian features and text description are input into the decoder, and the output is to generate the sentence probability distribution and hidden vector; then the semantic reconstruction network is established and the semantic reconstruction loss is calculated; using The stochastic gradient descent method optimizes the video description model composed of encoder, decoder and semantic reconstruction network; for new videos, t...
PUM
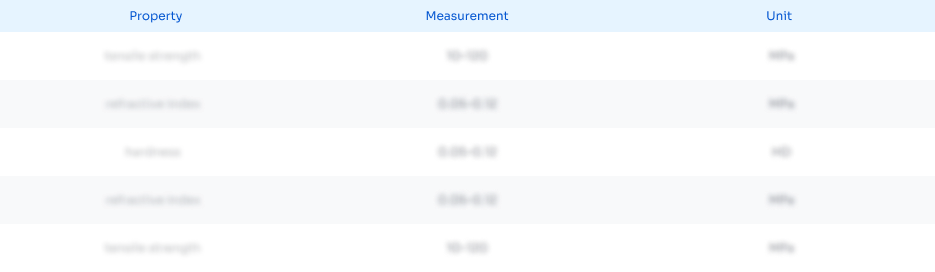
Abstract
Description
Claims
Application Information
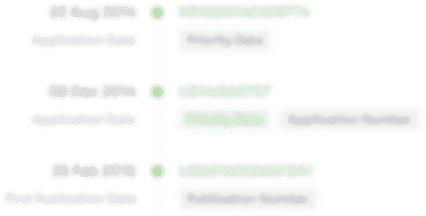
- R&D
- Intellectual Property
- Life Sciences
- Materials
- Tech Scout
- Unparalleled Data Quality
- Higher Quality Content
- 60% Fewer Hallucinations
Browse by: Latest US Patents, China's latest patents, Technical Efficacy Thesaurus, Application Domain, Technology Topic, Popular Technical Reports.
© 2025 PatSnap. All rights reserved.Legal|Privacy policy|Modern Slavery Act Transparency Statement|Sitemap|About US| Contact US: help@patsnap.com