Power load prediction method based on modular recurrent neural network
A technology of cyclic neural network and power load, applied in neural learning methods, biological neural network models, prediction, etc., can solve problems such as complex hidden layers and increased network parameters
- Summary
- Abstract
- Description
- Claims
- Application Information
AI Technical Summary
Problems solved by technology
Method used
Image
Examples
Embodiment Construction
[0065] The technical solution of the present invention will be specifically described below in conjunction with the accompanying drawings.
[0066] Such as figure 1 As shown, the present invention provides a kind of electric load forecasting method based on modular recurrent neural network, comprising the following steps:
[0067] Step S1, constructing a recurrent neural network comprising an input layer, a hidden layer and an output layer, and dividing the hidden layer into several modules;
[0068] Step S2, selecting any update strategy among fixed strategy, random strategy, disordered adaptive strategy and ordered adaptive strategy to update the hidden layer module;
[0069] Step S3, select the circular connection pruning strategy that matches the update strategy in step S2, wherein the fixed strategy and the ordered adaptive strategy can choose a unidirectional pruning strategy, while the random strategy and the disordered adaptive strategy can choose a bidirectional prun...
PUM
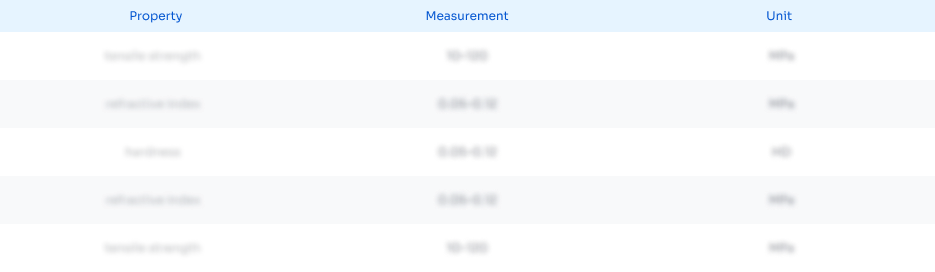
Abstract
Description
Claims
Application Information
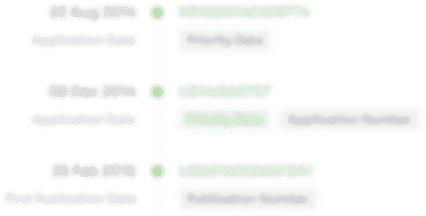
- R&D Engineer
- R&D Manager
- IP Professional
- Industry Leading Data Capabilities
- Powerful AI technology
- Patent DNA Extraction
Browse by: Latest US Patents, China's latest patents, Technical Efficacy Thesaurus, Application Domain, Technology Topic, Popular Technical Reports.
© 2024 PatSnap. All rights reserved.Legal|Privacy policy|Modern Slavery Act Transparency Statement|Sitemap|About US| Contact US: help@patsnap.com