Vehicle control dynamic imitation learning algorithm based on surround vision
A vehicle control and learning algorithm technology, applied in design optimization/simulation, instrumentation, electrical digital data processing, etc., can solve problems such as difficult to obtain dynamic obstacle motion state
- Summary
- Abstract
- Description
- Claims
- Application Information
AI Technical Summary
Problems solved by technology
Method used
Image
Examples
Embodiment Construction
[0062] Since the model is an end-to-end model based on surround vision, in order to enhance the generalization ability of the model and speed up the convergence of the loss function, the data in the dataset is preprocessed.
[0063] The unit of vehicle speed in the data set is m / s, and the vehicle speed distribution is as follows Figure 8 As shown, the horizontal axis of the coordinates in the figure is the vehicle speed, and the vertical axis is the number of samples. It can be seen that the vehicle speed of most samples does not exceed 11m / s, so all the vehicle speeds are divided by 12 for normalization, so that the normalized vehicle speed distribution is in the range of 0 to 1.
[0064]In order to cope with the challenge of different lighting environments on the visual model, the conventional method is to inject noise into the pictures in the data set with a certain probability. The transformation of the pictures in the experiment of the present invention refers to the da...
PUM
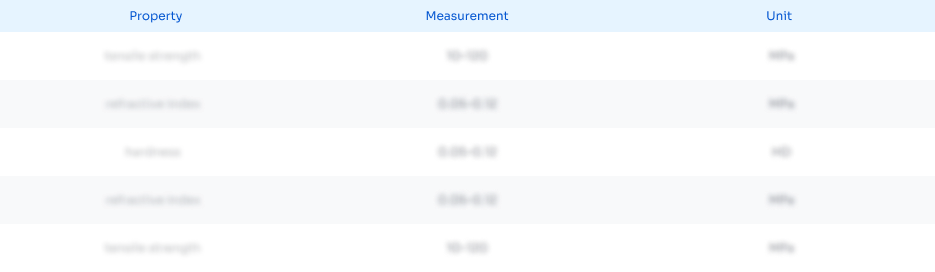
Abstract
Description
Claims
Application Information
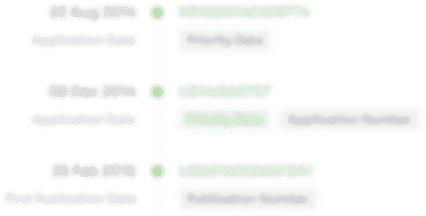
- R&D Engineer
- R&D Manager
- IP Professional
- Industry Leading Data Capabilities
- Powerful AI technology
- Patent DNA Extraction
Browse by: Latest US Patents, China's latest patents, Technical Efficacy Thesaurus, Application Domain, Technology Topic, Popular Technical Reports.
© 2024 PatSnap. All rights reserved.Legal|Privacy policy|Modern Slavery Act Transparency Statement|Sitemap|About US| Contact US: help@patsnap.com