Self-adaptive super-resolution method based on meta transfer learning
A technology of super-resolution and transfer learning, applied in neural learning methods, image analysis, image data processing, etc., can solve problems such as poor model performance, difficult model reproduction, ignoring the computational burden of deep neural networks, etc., and achieve generalization ability Strong, good reconstruction effect
- Summary
- Abstract
- Description
- Claims
- Application Information
AI Technical Summary
Problems solved by technology
Method used
Image
Examples
Embodiment Construction
[0032] The specific implementation manners of the present application will be further described below in conjunction with the accompanying drawings.
[0033] Such as figure 1 As shown, the adaptive super-resolution method based on meta-transfer learning involved in this application includes the following steps:
[0034] Step 1. Pre-train the adaptive super-resolution model based on meta-transfer learning through the external image data set, so that the model can initially learn the basic prior information of image reconstruction, that is, initially learn the common features of the image, and provide a basis for transfer Learning lays the foundation. Among them, the external image dataset refers to some recognized image collections in the field of image processing.
[0035] For the pre-training process, the preprocessing of the external image dataset is the same as the conventional model using bicubic interpolation to construct image pairs, and the adaptive super-resolution m...
PUM
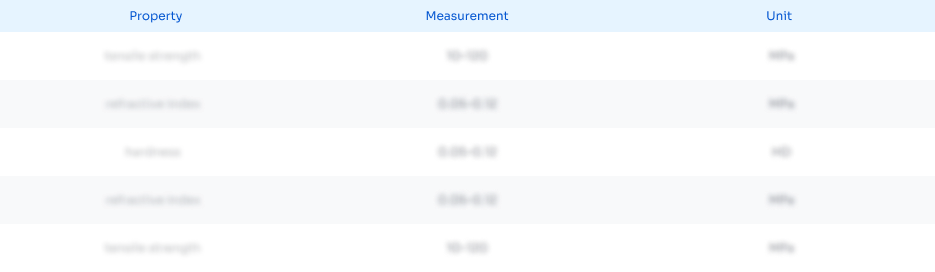
Abstract
Description
Claims
Application Information
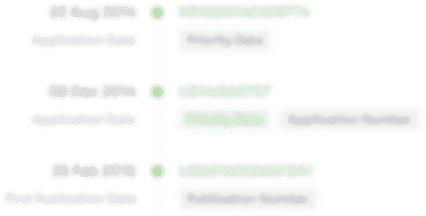
- R&D Engineer
- R&D Manager
- IP Professional
- Industry Leading Data Capabilities
- Powerful AI technology
- Patent DNA Extraction
Browse by: Latest US Patents, China's latest patents, Technical Efficacy Thesaurus, Application Domain, Technology Topic.
© 2024 PatSnap. All rights reserved.Legal|Privacy policy|Modern Slavery Act Transparency Statement|Sitemap