Deep learning-based stationary orbit meteorological satellite radar reflectivity factor inversion method
A radar reflection, geostationary orbit technology, applied in neural learning methods, reflection/re-radiation of radio waves, computer systems based on knowledge-based patterns, etc. and other problems, to achieve the effect of shortening the time interval, strong nonlinear reconstruction, and high inversion accuracy
- Summary
- Abstract
- Description
- Claims
- Application Information
AI Technical Summary
Problems solved by technology
Method used
Image
Examples
Embodiment 1
[0046] Such as figure 1 As shown, Embodiment 1 of the present invention proposes a method for inversion of geostationary satellite radar reflectivity factors based on deep learning. The invention is mainly composed of two modules, namely the preprocessing and training module on the left side of the block diagram and the verification module of the inventive technology shown on the right side of the block diagram.
[0047] 1. Data preparation
[0048] Firstly, the random forest method is used innovatively to select the channel with strong correlation with the radar reflectivity factor as the input of the model for the new generation geostationary orbit meteorological satellite imager data. On the one hand, it reduces the calculation and storage pressure, and on the other hand, it also clarifies the physical relationship between the radar reflectivity factor and the input. The radar reflectivity factor is closely related to the microphysical properties of clouds and the distrib...
Embodiment 2
[0068] Embodiment 2 of the present invention proposes a radar reflectivity factor inversion system for geostationary meteorological satellites based on deep learning. The system includes: a radar reflectivity factor inversion model, a sensitivity channel selection module, and an inversion result output module; the specific processing method is the same as in embodiment 1, wherein,
[0069] The sensitive channel selection module is used to select the sensitive channel of the geostationary meteorological satellite imager according to the random forest method; the sensitive channel is a channel with a strong correlation with the radar reflectivity factor;
[0070] The inversion result output module is used to receive the sensitive channel data transmitted by the imager of the geostationary meteorological satellite, input the pre-established and trained radar reflectivity factor inversion model, and obtain the inversion result.
PUM
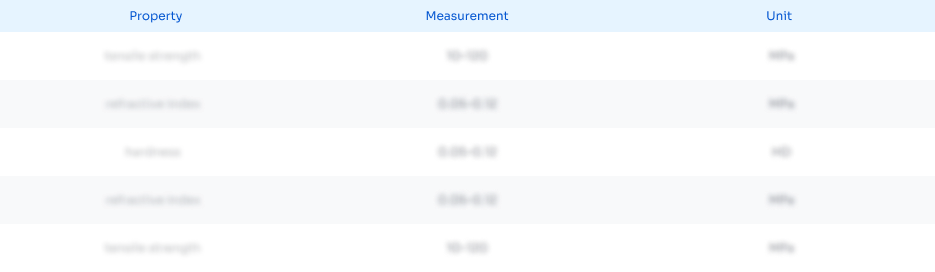
Abstract
Description
Claims
Application Information
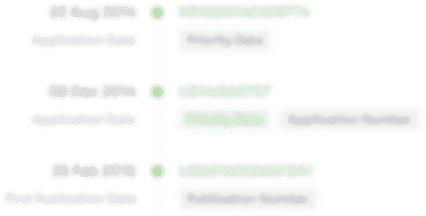
- R&D Engineer
- R&D Manager
- IP Professional
- Industry Leading Data Capabilities
- Powerful AI technology
- Patent DNA Extraction
Browse by: Latest US Patents, China's latest patents, Technical Efficacy Thesaurus, Application Domain, Technology Topic.
© 2024 PatSnap. All rights reserved.Legal|Privacy policy|Modern Slavery Act Transparency Statement|Sitemap