Federal learning global model training method based on differential privacy and quantification
A differential privacy and global model technology, applied in the field of data processing, can solve the problems of high communication cost, high computing overhead, privacy leakage, etc., and achieve the effect of improving communication efficiency and reducing communication bandwidth
- Summary
- Abstract
- Description
- Claims
- Application Information
AI Technical Summary
Problems solved by technology
Method used
Image
Examples
Embodiment Construction
[0033] Usually, federated learning uses private data distributed locally to perform distributed training to obtain a machine learning model with good predictive ability. Specifically, the central server obtains the global model gradient for updating the federated learning global model by aggregating local model gradients obtained by local users through local training. Then, the central server uses the global model gradient and the global model learning rate to update the federated learning global model. The federated learning global model update process is performed iteratively until a certain training termination condition is met.
[0034] The present invention will be further described in detail below in conjunction with the accompanying drawings and embodiments.
[0035] refer to figure 1 , to further describe in detail the implementation steps of the present invention.
[0036] Step 1. The central server delivers the pre-trained federated learning global model.
[0037] ...
PUM
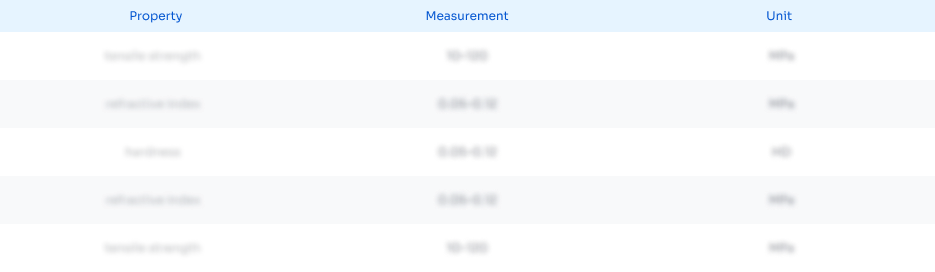
Abstract
Description
Claims
Application Information
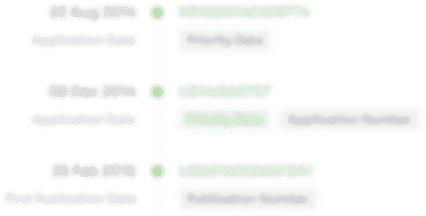
- R&D
- Intellectual Property
- Life Sciences
- Materials
- Tech Scout
- Unparalleled Data Quality
- Higher Quality Content
- 60% Fewer Hallucinations
Browse by: Latest US Patents, China's latest patents, Technical Efficacy Thesaurus, Application Domain, Technology Topic, Popular Technical Reports.
© 2025 PatSnap. All rights reserved.Legal|Privacy policy|Modern Slavery Act Transparency Statement|Sitemap|About US| Contact US: help@patsnap.com