Forgetting verification method based on semantic data loss in federated learning
A verification method and data technology, applied in semantic analysis, instrumentation, computing, etc., can solve problems such as forgetting verification and performance judgment, and achieve the effects of less time and space overhead, strong continuity, and good verification effect
- Summary
- Abstract
- Description
- Claims
- Application Information
AI Technical Summary
Problems solved by technology
Method used
Image
Examples
Embodiment Construction
[0022] The present invention will be described in further detail below in conjunction with the accompanying drawings and embodiments.
[0023] Such as figure 1 Shown is a schematic diagram of an instance of high-loss and error-prone semantic data describing a forgetting user in federated learning. Specifically, we show part of the data of the forgetting user. The first one represents the representative number normally classified as category "2" 2", and the rest are the actual category 7 with specific semantics that we screened out according to the loss and confidence distribution, and the specific classification and relabeling are samples of "2". It can be seen that these samples have an obvious features, that is, there is a horizontal line below the number, which is very close to the number "2" in shape, and by observing the output results of these special "7" after passing through the model, it can be found that these data have a relatively high probability is classified as...
PUM
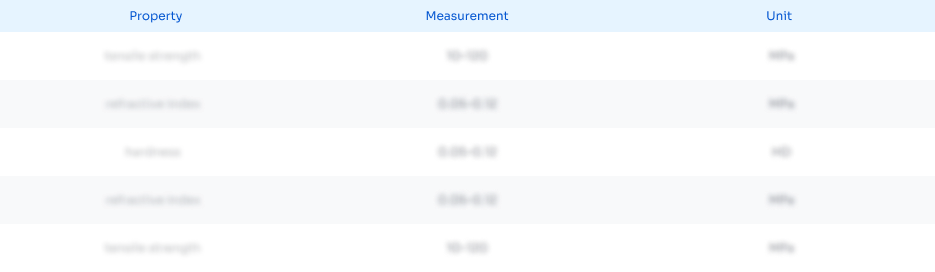
Abstract
Description
Claims
Application Information
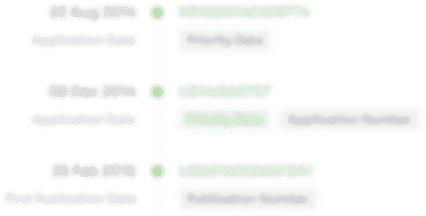
- R&D Engineer
- R&D Manager
- IP Professional
- Industry Leading Data Capabilities
- Powerful AI technology
- Patent DNA Extraction
Browse by: Latest US Patents, China's latest patents, Technical Efficacy Thesaurus, Application Domain, Technology Topic, Popular Technical Reports.
© 2024 PatSnap. All rights reserved.Legal|Privacy policy|Modern Slavery Act Transparency Statement|Sitemap|About US| Contact US: help@patsnap.com