ADMM-based unbalanced big data distributed classification method
A classification method and big data technology, applied in the optimization field of convex problems, can solve problems such as high time overhead and slow convergence speed, and achieve the effect of improving classification accuracy, speeding up calculation time, and alleviating class imbalance problems.
- Summary
- Abstract
- Description
- Claims
- Application Information
AI Technical Summary
Problems solved by technology
Method used
Image
Examples
Embodiment
[0106] Our method can solve a problem if it can be written as:
[0107] min x,y f(x)+g(y),
[0108] s.t.Ax+By=C,
[0109] Here f(x) and g(y) are both convex functions, and x and y are variables that satisfy a series of linear constraints. This way the dual of this problem can be solved. Its dual problem can be written in the following form:
[0110]
[0111] Here λ is the dual variable and ρ is the penalty coefficient. In particular, if the local variable x is partitionable, then f(x) can be partitioned into small problems stored on multiple machines. So the problem can be rewritten as follows:
[0112]
[0113] s.t.Ax i +By=C,i=1,2,...,n.
[0114] here x i is the model variable of the small problem on machine i, x=(x 1 ,...,x n ), y is a global variable.
PUM
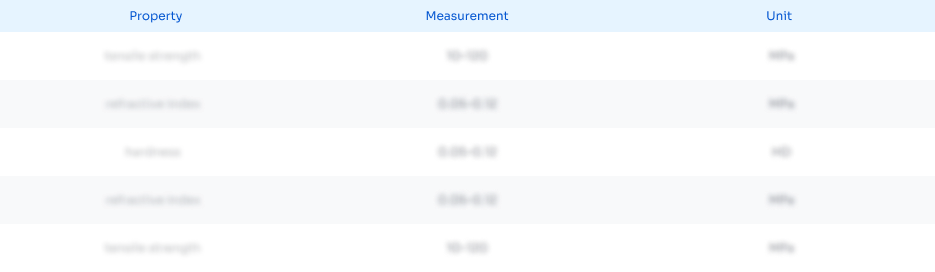
Abstract
Description
Claims
Application Information
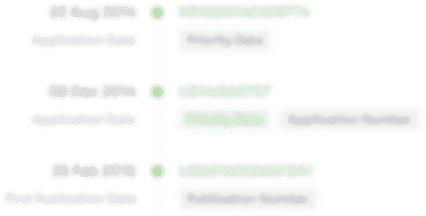
- Generate Ideas
- Intellectual Property
- Life Sciences
- Materials
- Tech Scout
- Unparalleled Data Quality
- Higher Quality Content
- 60% Fewer Hallucinations
Browse by: Latest US Patents, China's latest patents, Technical Efficacy Thesaurus, Application Domain, Technology Topic, Popular Technical Reports.
© 2025 PatSnap. All rights reserved.Legal|Privacy policy|Modern Slavery Act Transparency Statement|Sitemap|About US| Contact US: help@patsnap.com