Complex support vector machine classification method based on unitary space multi-feature fusion
A multi-feature fusion and support vector machine technology, which is applied to computer parts, instruments, calculations, etc., can solve the problem of low classification accuracy and achieve the effect of improving accuracy and reducing training and testing time
- Summary
- Abstract
- Description
- Claims
- Application Information
AI Technical Summary
Problems solved by technology
Method used
Image
Examples
Embodiment 1
[0051] Such as figure 1 Shown, in an embodiment of described classification method, comprise step:
[0052] S100. Extract a first feature and a second feature of an object to be classified.
[0053] The object to be classified can be an image, text, etc., and the first feature and the second feature are features extracted by different feature extraction methods, that is, the first feature is a feature extracted by the first feature extraction method, and the second feature is Using the features extracted by the second feature extraction method, taking the object to be classified as an example, the first feature can be an LBP (local binary pattern) feature, and the second feature can be a SURF (accelerated robust) feature, or, The first feature is the SURF feature, and the second feature is the LBP feature. As a global feature, the LBP feature is invariant to rotation and grayscale, but in some cases, such as when key parts are lost or occluded, the recognition rate is low. C...
Embodiment 2
[0127] Based on the above embodiments, the present invention also provides a corresponding classification device, such as Figure 10 As shown, the classification device includes:
[0128] An initial feature extraction module, the initial feature extraction module is used to extract the first feature and the second feature of the object to be classified, specifically as described in the first embodiment;
[0129] A feature fusion module, the feature fusion module is used to use the first feature of the object to be classified as a real part, and use the second feature of the object to be classified as an imaginary part to combine to obtain the complex feature corresponding to the object to be classified , specifically as described in Example 1;
[0130] A feature selection module, the feature selection module is used to perform feature selection on the complex features corresponding to the object to be classified according to a preset matrix, and extract the target feature cor...
Embodiment 3
[0133] Based on the above embodiments, the present invention also provides a corresponding terminal, such as Figure 11 As shown, the terminal includes a processor 10 and a memory 20 . Figure 11 Only some components of the terminal are shown, but it should be understood that implementation of all illustrated components is not required, and more or fewer components may be implemented instead.
[0134] The storage 20 may be an internal storage unit of the terminal in some embodiments, such as a hard disk or memory of the terminal. In other embodiments, the memory 20 may also be an external storage device of the terminal, such as a plug-in hard disk equipped on the terminal, a smart memory card (Smart Media Card, SMC), a secure digital (Secure Digital, SD ) card, flash memory card (Flash Card), etc. Further, the memory 20 may also include both an internal storage unit of the terminal and an external storage device. The memory 20 is used to store application software and vario...
PUM
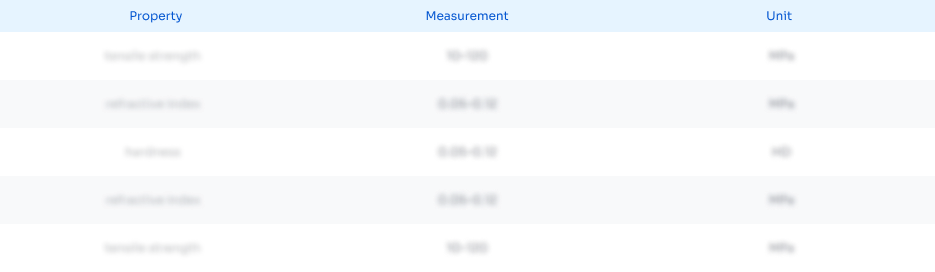
Abstract
Description
Claims
Application Information
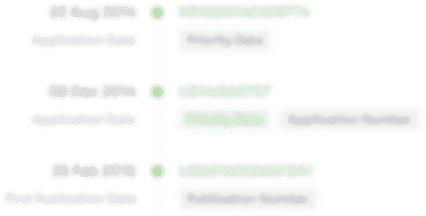
- R&D Engineer
- R&D Manager
- IP Professional
- Industry Leading Data Capabilities
- Powerful AI technology
- Patent DNA Extraction
Browse by: Latest US Patents, China's latest patents, Technical Efficacy Thesaurus, Application Domain, Technology Topic, Popular Technical Reports.
© 2024 PatSnap. All rights reserved.Legal|Privacy policy|Modern Slavery Act Transparency Statement|Sitemap|About US| Contact US: help@patsnap.com