Hypergraph neural network classification method and device
A neural network and classification method technology, applied in the field of complex network node classification, can solve problems such as low learning efficiency of high-order complex association models, and achieve the effect of reducing training speed and inference speed
- Summary
- Abstract
- Description
- Claims
- Application Information
AI Technical Summary
Problems solved by technology
Method used
Image
Examples
Embodiment Construction
[0044] Embodiments of the present application are described in detail below, and examples of the embodiments are shown in the drawings, wherein the same or similar reference numerals denote the same or similar elements or elements having the same or similar functions throughout. The embodiments described below by referring to the figures are exemplary, and are intended to explain the present application, and should not be construed as limiting the present application.
[0045] The hypergraph neural network classification method and device according to the embodiments of the present application are described below with reference to the accompanying drawings.
[0046] figure 1It is a flowchart of a hypergraph neural network classification method provided in Embodiment 1 of the present application.
[0047] Such as figure 1 As shown, the hypergraph neural network classification method includes the following steps:
[0048] Step 101, obtaining label data to be predicted;
[00...
PUM
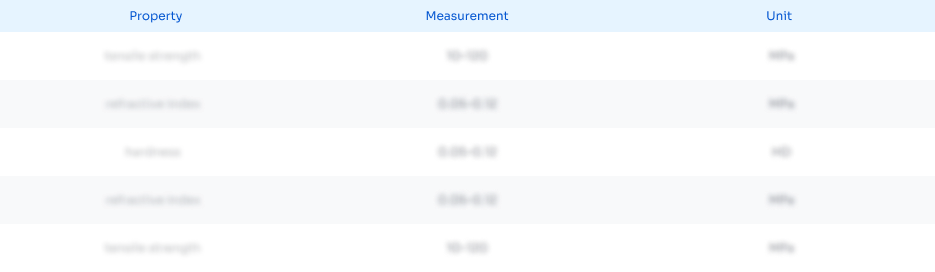
Abstract
Description
Claims
Application Information
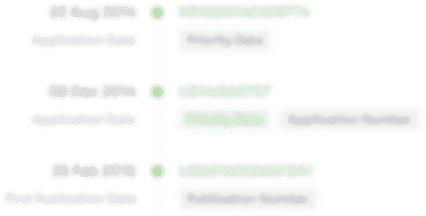
- R&D
- Intellectual Property
- Life Sciences
- Materials
- Tech Scout
- Unparalleled Data Quality
- Higher Quality Content
- 60% Fewer Hallucinations
Browse by: Latest US Patents, China's latest patents, Technical Efficacy Thesaurus, Application Domain, Technology Topic, Popular Technical Reports.
© 2025 PatSnap. All rights reserved.Legal|Privacy policy|Modern Slavery Act Transparency Statement|Sitemap|About US| Contact US: help@patsnap.com