Panorama-based self-supervised learning scene point cloud completion data set generation method
A supervised learning and point cloud completion technology, applied in the field of 3D reconstruction, can solve problems such as difficult to reconstruct real point cloud scenes, difficult to obtain data, and lack of integrity
- Summary
- Abstract
- Description
- Claims
- Application Information
AI Technical Summary
Problems solved by technology
Method used
Image
Examples
Embodiment Construction
[0042] The specific implementation manners of the present invention will be further described below in conjunction with the drawings and technical solutions.
[0043] The present invention is based on the 2D-3D-Semantics dataset released by Stanford University. This dataset involves 6 large indoor areas originating from 3 different buildings mainly education and office. The data set contains a total of 1413 equirectangular panoramic RGB images, as well as corresponding depth maps, surface normal maps, semantic annotation maps, and camera metadata, which are sufficient to support the self-supervised learning scene point cloud compensation based on panoramic images proposed by the present invention. A complete dataset generation method. In addition, other equirectangular panoramas taken or collected are also applicable to the present invention.
[0044] The present invention includes four main modules, which are respectively 2D-3D rectangular projection module, viewpoint selec...
PUM
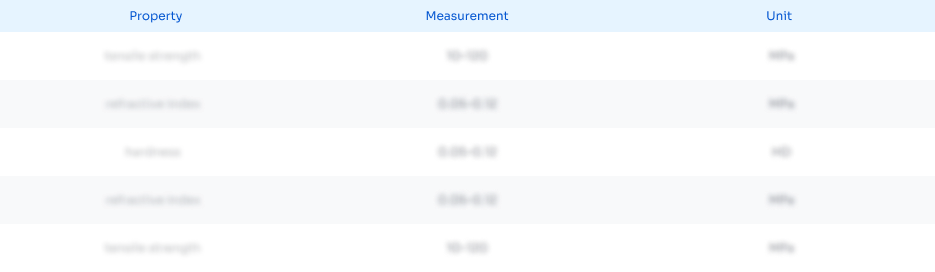
Abstract
Description
Claims
Application Information
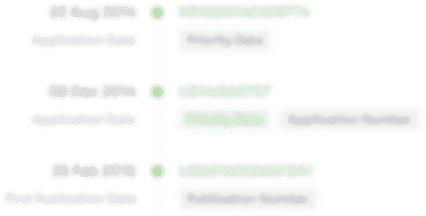
- R&D
- Intellectual Property
- Life Sciences
- Materials
- Tech Scout
- Unparalleled Data Quality
- Higher Quality Content
- 60% Fewer Hallucinations
Browse by: Latest US Patents, China's latest patents, Technical Efficacy Thesaurus, Application Domain, Technology Topic, Popular Technical Reports.
© 2025 PatSnap. All rights reserved.Legal|Privacy policy|Modern Slavery Act Transparency Statement|Sitemap|About US| Contact US: help@patsnap.com