Malicious software multi-label classification method based on graph convolutional neural network
A convolutional neural network and classification method technology, applied in the field of malware detection, can solve problems such as behavioral deviation, bad behavior, and not being able to represent the real behavior of samples, and achieve good classification results
- Summary
- Abstract
- Description
- Claims
- Application Information
AI Technical Summary
Problems solved by technology
Method used
Image
Examples
Embodiment Construction
[0062] In order to make the purpose, technical solutions and advantages of the embodiments of the present disclosure clearer, the technical solutions of the embodiments of the present disclosure will be clearly and completely described below in conjunction with the accompanying drawings of the embodiments of the present disclosure.
[0063] see Figure 1-3 Shown, is a kind of malware multi-label classification method based on graph convolutional neural network of the present invention, this malware multi-label classification method based on graph convolutional neural network comprises the following steps:
[0064] S100: feature extraction of the function call graph, disassemble the original binary file, obtain the assembly code, extract the semantic and structural features of the function call graph, and obtain the graph embedding vector of the sample;
[0065] S200: feature extraction of multi-label relationship, constructing a model for extracting label relationship through ...
PUM
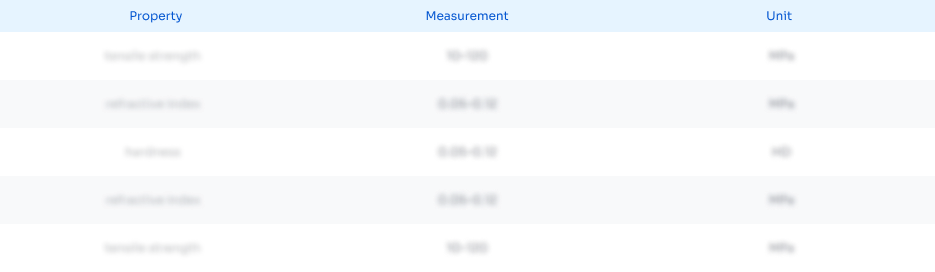
Abstract
Description
Claims
Application Information
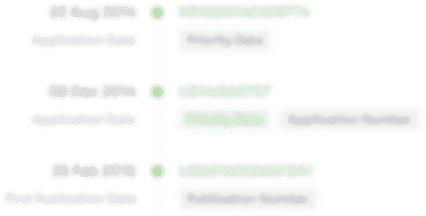
- R&D Engineer
- R&D Manager
- IP Professional
- Industry Leading Data Capabilities
- Powerful AI technology
- Patent DNA Extraction
Browse by: Latest US Patents, China's latest patents, Technical Efficacy Thesaurus, Application Domain, Technology Topic, Popular Technical Reports.
© 2024 PatSnap. All rights reserved.Legal|Privacy policy|Modern Slavery Act Transparency Statement|Sitemap|About US| Contact US: help@patsnap.com