Class-specified multi-mode joint representation method for large-scene remote sensing image classification
A technology for remote sensing images and large scenes, applied in the field of joint representation of multi-mode remote sensing images, to achieve good discrimination, improved classification performance, and high classification accuracy
- Summary
- Abstract
- Description
- Claims
- Application Information
AI Technical Summary
Problems solved by technology
Method used
Image
Examples
specific Embodiment approach 1
[0023] Specific implementation mode one: combine figure 1 This embodiment is described. In this embodiment, a class-specified multi-mode joint representation method for remote sensing image classification of large scenes is described in detail as follows:
[0024] Step 1. Input the multi-mode remote sensing image with the same coverage area, and the corresponding feature label map (both H and G in the formula are obtained based on this feature label map), and construct the class-specified multi-mode joint of the multi-mode remote sensing image Representation model, mainly including reconstruction error constraints, discriminative constraints and classification constraints;
[0025] The multimode remote sensing images include multispectral remote sensing images and hyperspectral remote sensing images;
[0026] The model optimization of step 2 and step 1 is based on the idea that the zero point of the first-order derivative of the constraint model is the extreme point, and the ...
specific Embodiment approach 2
[0031] Specific embodiment two: the difference between this embodiment and specific embodiment one is: in the described step one, construct the class-specified multi-mode joint representation model of the multi-mode remote sensing image; the specific process is:
[0032] make and Respectively represent the set of labeled samples in the hyperspectral and multispectral remote sensing images with the same coverage area of the input, and these samples are the samples of the class-specified multi-mode joint representation;
[0033] make and Represent hyperspectral dictionary and multispectral dictionary respectively;
[0034] Let X denote the cross-modal sparse representation coefficient matrix;
[0035] in, Represents a labeled sample in a hyperspectral image, Denotes a labeled sample in a multispectral image, d H Indicates the spectral dimension of the sample in the hyperspectral image, d M Indicates the spectral dimension of the sample in the multispectral image,...
specific Embodiment approach 3
[0041] Embodiment 3: The difference between this embodiment and Embodiment 1 or 2 is that the class of the multi-mode remote sensing image specifies the first two items in the objective function of the multi-mode joint representation model Represents the reconstruction error, the third term α||X|| 1,1 Represents the sparsity constraint of the cross-mode sparse representation coefficient, the fourth term β(||D H || * +||D M || * ) represents the low-rank constraint of the cross-modulus dictionary, and the fifth item Represents discriminative constraints, the sixth term Represents a classification constraint.
[0042] Other steps are the same as those in Embodiment 1 or 2.
PUM
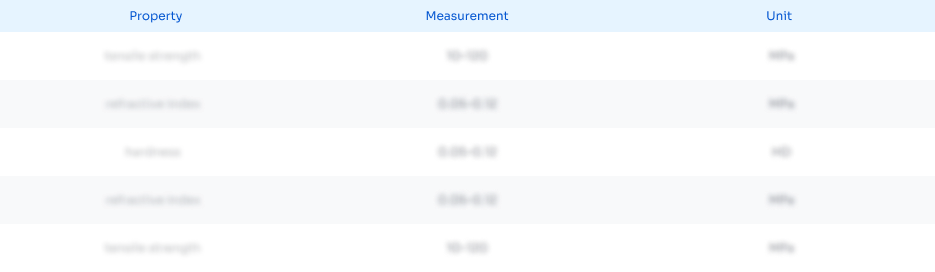
Abstract
Description
Claims
Application Information
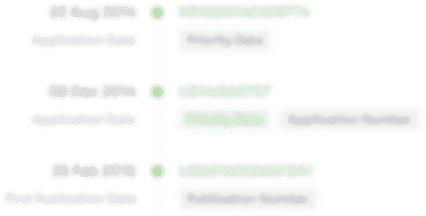
- R&D
- Intellectual Property
- Life Sciences
- Materials
- Tech Scout
- Unparalleled Data Quality
- Higher Quality Content
- 60% Fewer Hallucinations
Browse by: Latest US Patents, China's latest patents, Technical Efficacy Thesaurus, Application Domain, Technology Topic, Popular Technical Reports.
© 2025 PatSnap. All rights reserved.Legal|Privacy policy|Modern Slavery Act Transparency Statement|Sitemap|About US| Contact US: help@patsnap.com