Abnormal group detection method based on unsupervised algorithm
An unsupervised, group technology, applied in the field of electronic information, can solve problems such as inability to perceive new risk types in advance, inability to deal with black production attack methods, lack of interpretability, etc., to facilitate identification and disposal, and save manual annotation. cost, effect of increasing interpretability
- Summary
- Abstract
- Description
- Claims
- Application Information
AI Technical Summary
Problems solved by technology
Method used
Image
Examples
Embodiment 1
[0033] Such as Figure 1-2 , the present invention provides an anomaly group detection method based on an unsupervised algorithm. Firstly, the user’s transaction data and operation data are integrated for data preprocessing, and relevant model features are selected according to business experience, and then the weight of different features and the relationship between samples are determined. A measure of similarity to group users using an unsupervised clustering algorithm. Then use the FP-growth frequent set mining algorithm to analyze the aggregation reason and aggregation degree of each group. Finally, combined with the clustering degree and risk degree of each group, a comprehensive risk score is given to the group. The higher the risk score, the greater the risk of the group.
[0034] figure 1 It is a flow chart of the abnormal group detection method based on the unsupervised algorithm shown according to the implementation process of the example, refer to figure 1 As sh...
PUM
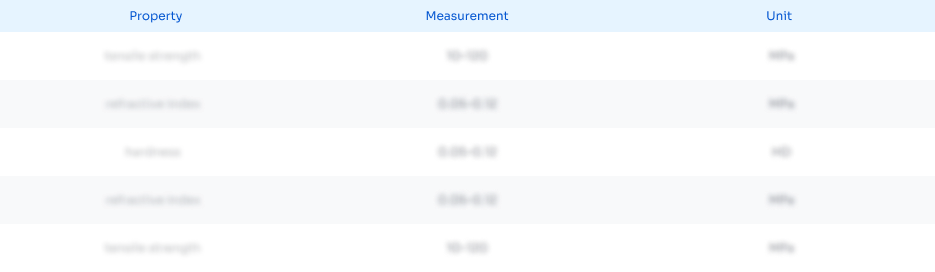
Abstract
Description
Claims
Application Information
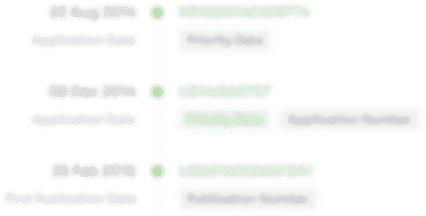
- R&D
- Intellectual Property
- Life Sciences
- Materials
- Tech Scout
- Unparalleled Data Quality
- Higher Quality Content
- 60% Fewer Hallucinations
Browse by: Latest US Patents, China's latest patents, Technical Efficacy Thesaurus, Application Domain, Technology Topic, Popular Technical Reports.
© 2025 PatSnap. All rights reserved.Legal|Privacy policy|Modern Slavery Act Transparency Statement|Sitemap|About US| Contact US: help@patsnap.com