Unsupervised cross-modal retrieval method based on attention mechanism enhancement
An attention-based, cross-modal technology, applied in the field of artificial intelligence smart community applications, can solve the problems of indirect increase in the heterogeneous semantic gap, unequal semantic information, failure to retrieve data of different modalities, etc., to achieve rich visual semantics Information, Robust Representation, Effects of Closing the Semantic Gap
- Summary
- Abstract
- Description
- Claims
- Application Information
AI Technical Summary
Problems solved by technology
Method used
Image
Examples
Embodiment
[0034] The workflow of the embodiment of the present invention is as figure 1 As shown, it mainly includes the following seven parts:
[0035] (1) Preprocess the image data and text data, and change the size of the image data to 224 224, cut the picture into nine pieces; for text data, turn it into a word vector of the corresponding dimension;
[0036] (2) Perform feature extraction on the image and text data processed in step (1), input the processed image into the attention mechanism network, use the self-attention module to perform feature extraction, obtain image features, and form image feature vectors Collection; text data uses linear layer for further feature extraction to form a collection of text feature vectors;
[0037] (3) Input the image and text feature vector sets extracted in step (2) into the multimodal feature fusion module, that is, first fuse the extracted image and text feature vector sets on the 512-dimensional intermediate dimension to obtain multimod...
PUM
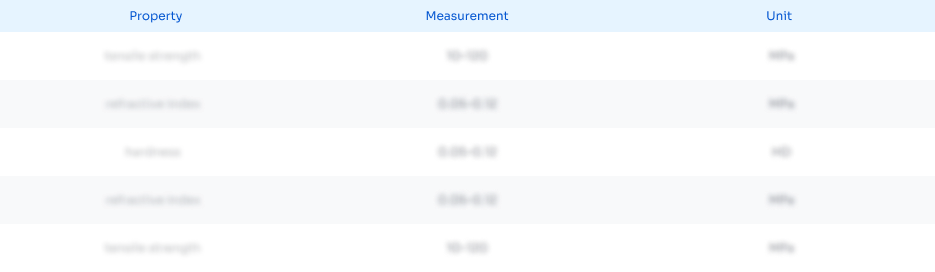
Abstract
Description
Claims
Application Information
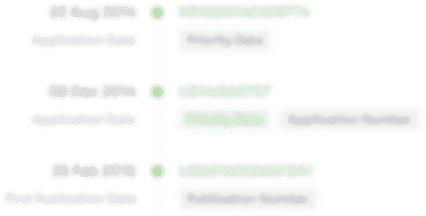
- R&D Engineer
- R&D Manager
- IP Professional
- Industry Leading Data Capabilities
- Powerful AI technology
- Patent DNA Extraction
Browse by: Latest US Patents, China's latest patents, Technical Efficacy Thesaurus, Application Domain, Technology Topic, Popular Technical Reports.
© 2024 PatSnap. All rights reserved.Legal|Privacy policy|Modern Slavery Act Transparency Statement|Sitemap|About US| Contact US: help@patsnap.com