Digital media protection text steganography method based on variational automatic encoder
A digital media protection and autoencoder technology, applied in the field of information security, can solve the problems of limited text usage scenarios, uncontrollable text content, unsupervised and other problems, and achieve the effect of visual indistinguishability
- Summary
- Abstract
- Description
- Claims
- Application Information
AI Technical Summary
Problems solved by technology
Method used
Image
Examples
Embodiment 1
[0069] In this embodiment, the digital media protection text steganography method based on variational autoencoder is as follows figure 1 , the global keywords, long sequences and secret information to be hidden are taken as input data packets, and the output after the steganographic text generation model is steganographic text embedded with secret information. Specifically, it includes the following four steps:
[0070] S1: Data preprocessing. In the data preprocessing part, it is necessary to extract the global keywords and group keywords of the training text, and it is necessary to divide a long sequence into multiple short sequences, and each short sequence corresponds to a group of keywords, and the global keywords are all A union of group keywords. If you want to embed secret information in plain text, you also need to encode the secret information into a bit stream.
[0071] S2: Extract textual context relevance. First of all, it is necessary to process the text int...
Embodiment 2
[0075] This embodiment further explains the data preprocessing of step S1 in embodiment 1. This step is mainly divided into the following four steps:
[0076] S11: Sequence word segmentation. Usually, keywords cannot be obtained directly from the original sequence. Words that have nothing to do with part of speech usually affect the accuracy of keywords. Stop words are removed from the word segmentation results.
[0077] S12: acquisition of global keywords and group keywords. Group keywords are mainly used for local control of text generation content, and mature keyword extraction tools are usually used to obtain text keywords; global keywords are the union of group keywords.
[0078] S13: long sequence division. Using long sequences for text generation often loses relevant contextual features in the second half of the text, and may lead to uncontrollable content of text generation. Therefore, it is necessary to divide the long text into multiple short sequences, and then t...
Embodiment 3
[0104] This embodiment further describes the neural network model constructed by the present invention. The model is mainly divided into three stages: encoding network, Gaussian sampling, and decoding network. The first stage of the encoding network is mainly to fuse global keywords and long sequences to obtain its global feature representation; the second stage of Gaussian sampling is mainly used to perform Gaussian sampling on the global features in the encoding network, and the sampling results obey the isotropic Gaussian distribution; the second stage The three-stage decoding network mainly decodes the sampling results, and then realizes the steganography of the text according to the conditional probability distribution of the text and combined with the corresponding encoding algorithm. For the encoding network, Gaussian sampling, and decoding network, it specifically includes the following steps:
[0105] S31: Coding Network
[0106] The encoding network is divided into ...
PUM
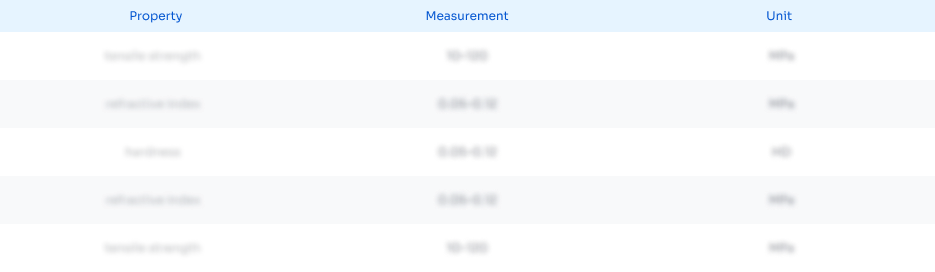
Abstract
Description
Claims
Application Information
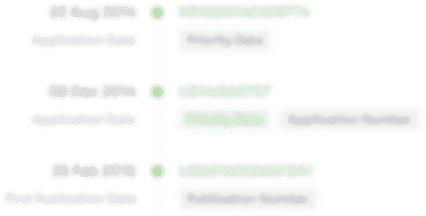
- Generate Ideas
- Intellectual Property
- Life Sciences
- Materials
- Tech Scout
- Unparalleled Data Quality
- Higher Quality Content
- 60% Fewer Hallucinations
Browse by: Latest US Patents, China's latest patents, Technical Efficacy Thesaurus, Application Domain, Technology Topic, Popular Technical Reports.
© 2025 PatSnap. All rights reserved.Legal|Privacy policy|Modern Slavery Act Transparency Statement|Sitemap|About US| Contact US: help@patsnap.com