Underwater unmanned vehicle safety opportunistic routing method and device based on reinforcement learning
An unmanned vehicle and reinforcement learning technology, applied in the safety opportunity routing of underwater unmanned vehicles, the field of safety opportunity routing of underwater unmanned vehicles based on reinforcement learning, can solve small topology changes, empty nodes, and can not be autonomous Mobile and other issues, to achieve the effect of optimizing overall performance, achieving security and efficiency, and avoiding effective transmission
- Summary
- Abstract
- Description
- Claims
- Application Information
AI Technical Summary
Problems solved by technology
Method used
Image
Examples
Embodiment 1
[0053] Embodiment one, see figure 1 This embodiment will be described. A safety opportunity routing method for underwater unmanned vehicles based on reinforcement learning described in this embodiment, the method includes:
[0054] The underwater unmanned vehicle is initially screened within the node-to-communication range, and a trust evaluation model is established based on the initially screened nodes;
[0055] Establish a trust evaluation model based on the initially screened nodes for evaluation, the evaluation elements of the evaluation model are composed of two parts: direct trust value DTValue and indirect trust value ITValue;
[0056] The evaluation elements are input into the fuzzy logic system to obtain the comprehensive trust value of the evaluation node, and the comprehensive trust value of the evaluation node is updated to the dynamic table of the trust value of the meeting node;
[0057] According to the comprehensive trust value of evaluation nodes output by ...
Embodiment 2
[0059] Embodiment two, see figure 1 This embodiment will be described. This embodiment is a further limitation of the safety opportunity routing method for underwater unmanned vehicles based on reinforcement learning described in the first embodiment. In this embodiment, the underwater unmanned vehicles are within the node-to-node communication range The process of initial screening and establishing a trust evaluation model based on the initially screened nodes is as follows:
[0060] The underwater unmanned vehicle node carrying the message sends a broadcast to other nodes within the communication range, requests other nodes to feed back their node information, obtains data packets, performs initial screening according to the indirect trust value ITValue in the other party's data packet information, and selects the indirect trust value Nodes exceeding the threshold are further evaluated as candidate relay nodes.
[0061] In this embodiment, node information is obtained by p...
Embodiment 3
[0062] Embodiment three, refer to figure 2 This embodiment will be described. This embodiment is a further limitation of the safety opportunity routing method for underwater unmanned vehicles based on reinforcement learning described in Embodiment 1. In this embodiment, the evaluation elements of the direct trust value DTValue are selected as: 1. The relative distance between nodes is calculated by the time difference between sending and receiving node data packets, and the path loss is estimated by the relative distance between nodes to measure the communication quality between nodes; 2. Node familiarity; 3. Node relay ratio.
[0063] The indirect trust value DTValue guarantees the objectivity of the evaluation of the current node, each node maintains a dynamic trust value table, records the comprehensive trust value data of other nodes to itself, and the average value of the data in the dynamic trust value table is output as the indirect trust value .
[0064] In this emb...
PUM
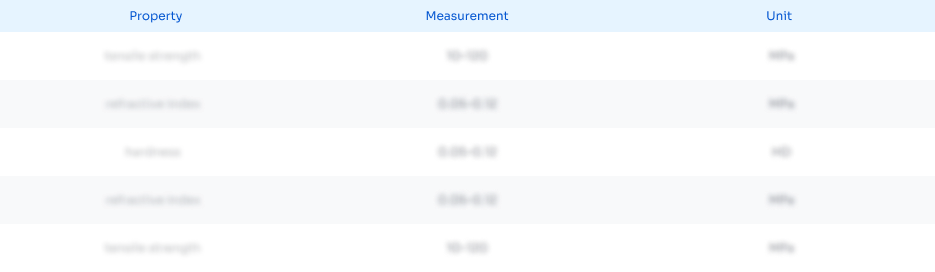
Abstract
Description
Claims
Application Information
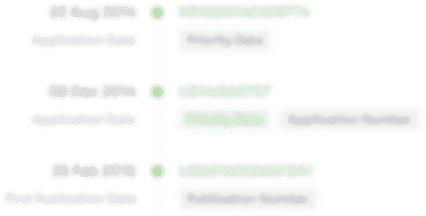
- R&D Engineer
- R&D Manager
- IP Professional
- Industry Leading Data Capabilities
- Powerful AI technology
- Patent DNA Extraction
Browse by: Latest US Patents, China's latest patents, Technical Efficacy Thesaurus, Application Domain, Technology Topic, Popular Technical Reports.
© 2024 PatSnap. All rights reserved.Legal|Privacy policy|Modern Slavery Act Transparency Statement|Sitemap|About US| Contact US: help@patsnap.com