Mobile cellular traffic efficient prediction method based on space-time aggregation graph convolutional network
A convolutional network and mobile cellular technology, applied in the field of mobile communications, can solve the problems of complex temporal and spatial dependence of mobile traffic in the city and a large number of resources
- Summary
- Abstract
- Description
- Claims
- Application Information
AI Technical Summary
Problems solved by technology
Method used
Image
Examples
Embodiment Construction
[0052] The present invention provides a high-efficiency prediction method for cellular traffic based on spatio-temporal aggregated graph convolutional network. The whole model consists of four modules, namely aggregated graph convolutional network module, embedding module, regression module and external module. First, the aggregated graph convolutional network divides the prediction area into multiple sub-regions and regards them as individual nodes in the network, by modeling the daily historical patterns and hourly current patterns of mobile cellular traffic, capturing the data across all nodes at different times. Complex spatiotemporal correlations. Next, the embedding module concatenates the outputs of the K-layer aggregated graph convolutional network modules. Then, the regression module fuses the prediction information with the external features extracted by the external module to obtain the final mobile cellular traffic prediction result, and updates the model parameter...
PUM
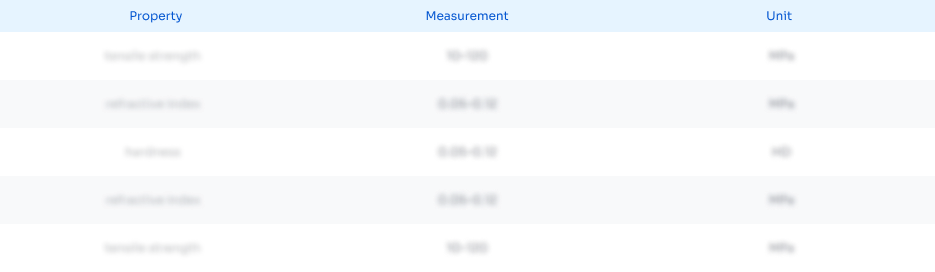
Abstract
Description
Claims
Application Information
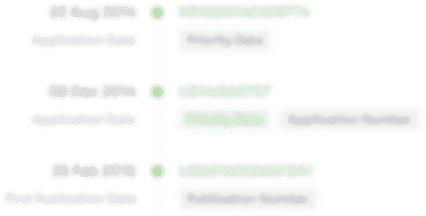
- Generate Ideas
- Intellectual Property
- Life Sciences
- Materials
- Tech Scout
- Unparalleled Data Quality
- Higher Quality Content
- 60% Fewer Hallucinations
Browse by: Latest US Patents, China's latest patents, Technical Efficacy Thesaurus, Application Domain, Technology Topic, Popular Technical Reports.
© 2025 PatSnap. All rights reserved.Legal|Privacy policy|Modern Slavery Act Transparency Statement|Sitemap|About US| Contact US: help@patsnap.com