Gastric cancer TNM staging prediction system based on multi-modal deep learning
A deep learning and prediction system technology, applied in the field of medical artificial intelligence, can solve problems such as insufficient processing tasks, and achieve the effect of reducing the workload of reading images and improving medical interpretability
- Summary
- Abstract
- Description
- Claims
- Application Information
AI Technical Summary
Problems solved by technology
Method used
Image
Examples
Embodiment Construction
[0029] The present invention will be further described in detail below with reference to the accompanying drawings and embodiments. It should be noted that the following embodiments are intended to facilitate the understanding of the present invention, but do not limit it in any way.
[0030] A gastric cancer TNM staging prediction system based on multimodal deep learning, comprising a computer memory, a computer processor, and a computer program stored in the computer memory and executable on the computer processor, the computer memory is stored with a data acquisition module, an image Preprocessing module, text preprocessing module, predictive model training module and prediction module. The specific application process of each module is introduced in detail below.
[0031] 1. Data acquisition module
[0032] The desensitized data of 284 patients with gastric cancer in the imaging department of the hospital were collected. In addition to computed tomography (CT) images, the...
PUM
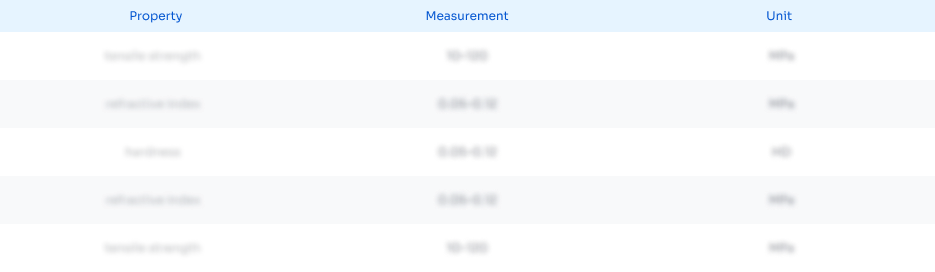
Abstract
Description
Claims
Application Information
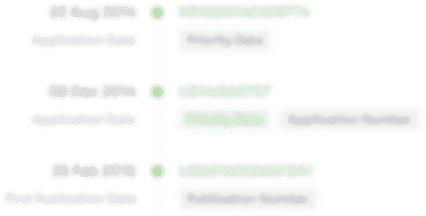
- R&D Engineer
- R&D Manager
- IP Professional
- Industry Leading Data Capabilities
- Powerful AI technology
- Patent DNA Extraction
Browse by: Latest US Patents, China's latest patents, Technical Efficacy Thesaurus, Application Domain, Technology Topic, Popular Technical Reports.
© 2024 PatSnap. All rights reserved.Legal|Privacy policy|Modern Slavery Act Transparency Statement|Sitemap|About US| Contact US: help@patsnap.com