Semi-automatic demand extraction method based on pre-training language fine tuning and dependency features
A pre-training and semi-automatic technology, applied in the field of information extraction of natural language processing, can solve problems such as opaque capture rules of BERT, poor interpretability of deep learning, time-consuming BERT model training, etc., to improve interpretability and reliability Effect
- Summary
- Abstract
- Description
- Claims
- Application Information
AI Technical Summary
Problems solved by technology
Method used
Image
Examples
Embodiment Construction
[0027] A brief overview of the invention is given below in order to provide a basic understanding of some aspects of the invention. It should be understood that this summary is not an exhaustive overview of the invention. It is not intended to identify key or critical parts of the invention nor to delineate the scope of the invention. Its purpose is merely to present some concepts in a simplified form as a prelude to the more detailed description that is discussed later.
[0028] Such as figure 1 As shown, the semi-automatic requirement extraction method includes the following steps: preprocessing step, entity extraction step, entity fusion confirmation step, intent extraction step, intent fusion confirmation step, subject relationship post-processing step and output modeling. For the components required by the iStar target model, the subject (Actor) and resource (Resource) are processed in the entity extraction, the dependency between the intent elements such as the task (Tas...
PUM
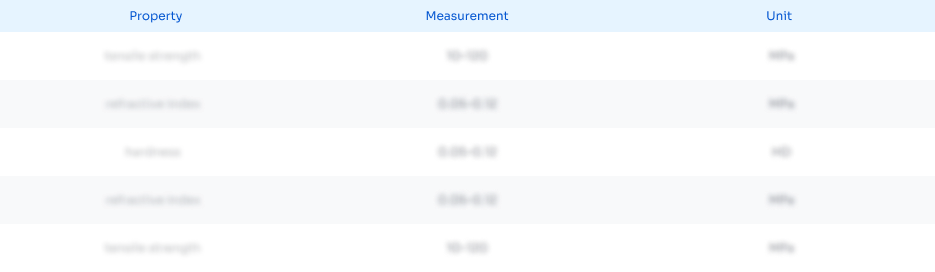
Abstract
Description
Claims
Application Information
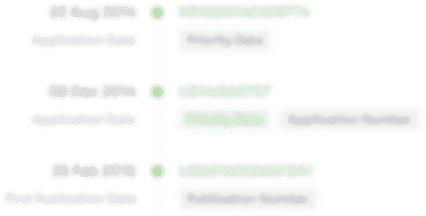
- R&D Engineer
- R&D Manager
- IP Professional
- Industry Leading Data Capabilities
- Powerful AI technology
- Patent DNA Extraction
Browse by: Latest US Patents, China's latest patents, Technical Efficacy Thesaurus, Application Domain, Technology Topic, Popular Technical Reports.
© 2024 PatSnap. All rights reserved.Legal|Privacy policy|Modern Slavery Act Transparency Statement|Sitemap|About US| Contact US: help@patsnap.com