Training method, system, device and storage medium for domain-adapted image classification network
A classification network and training method technology, applied in biological neural network models, instruments, calculations, etc., can solve the problems of large model parameters, long training time, training efficiency limitations, etc., achieve accurate image classification results, improve accuracy, The effect of improving overall performance
- Summary
- Abstract
- Description
- Claims
- Application Information
AI Technical Summary
Problems solved by technology
Method used
Image
Examples
Embodiment 1
[0036] The embodiment of the present invention provides a training method for a domain-adapted image classification network. Different from the existing scheme in which the feature before the classifier is directly used to calculate the contrast loss, the present invention uses the probability after the classifier to calculate the probability contrast loss, so as to facilitate the clustering process. It effectively constrains the class weights and the distance between features while keeping the features of the same class. Specifically, the present invention transfers the contrastive learning from the feature space to the probability space, and removes thel 2 Norm normalization to constrain the probability to take on a one-hot form. like figure 1 As shown, the method provided by the invention mainly comprises the following steps:
[0037] Step 1. Obtain the source domain image set, and obtain the target domain image set according to the training method; perform two different ...
Embodiment 2
[0102] The present invention also provides a training system for a domain-adapted image classification network, which is mainly implemented based on the method provided in the first embodiment, such as Figure 5 As shown, the system mainly includes:
[0103] The training data set construction unit is used to obtain the source domain image set, and obtain the target domain image set according to the training method; perform two different image transformations on each unlabeled target domain image in the target domain image set, and obtain the first The transformed image and the second transformed image form a target domain image pair, and a training data set is formed by the source domain image set, the target domain image set and all target domain image pairs;
[0104] a training data set input unit for inputting the training data set to the domain-adapted image classification network;
[0105] The baseline loss calculation unit is used to calculate one or more of the output ...
Embodiment 3
[0111] The present invention also provides a processing device, such as Image 6 As shown, it mainly includes: one or more processors; a memory for storing one or more programs; wherein, when the one or more programs are executed by the one or more processors, the One or more processors implement the methods provided by the foregoing embodiments.
[0112] Further, the processing device further includes at least one input device and at least one output device; in the processing device, the processor, the memory, the input device, and the output device are connected through a bus.
[0113] In this embodiment of the present invention, the specific types of the memory, input device, and output device are not limited; for example:
[0114] The input device can be a touch screen, an image capture device, a physical button or a mouse, etc.;
[0115] The output device can be a display terminal;
[0116] The memory may be random access memory (Random Access Memory, RAM), or may be non...
PUM
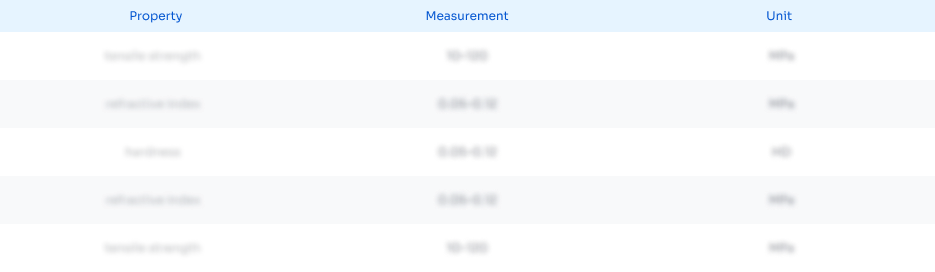
Abstract
Description
Claims
Application Information
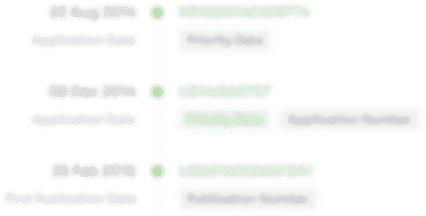
- R&D
- Intellectual Property
- Life Sciences
- Materials
- Tech Scout
- Unparalleled Data Quality
- Higher Quality Content
- 60% Fewer Hallucinations
Browse by: Latest US Patents, China's latest patents, Technical Efficacy Thesaurus, Application Domain, Technology Topic, Popular Technical Reports.
© 2025 PatSnap. All rights reserved.Legal|Privacy policy|Modern Slavery Act Transparency Statement|Sitemap|About US| Contact US: help@patsnap.com