An Active Sampling Method for Target Detection Based on Timing Variance Threshold
A target detection and active sampling technology, which is applied in the direction of instruments, computing, character and pattern recognition, etc., can solve the problems of high cost and loss of key information, and achieve the effect of reducing negative impact and labeling cost
- Summary
- Abstract
- Description
- Claims
- Application Information
AI Technical Summary
Problems solved by technology
Method used
Image
Examples
Embodiment
[0041] like figure 1 Shown is a flow diagram of the mechanism of the present invention. It is assumed that initially there is a data set L composed of a small number of labeled time series data images, and a data set U composed of a large number of unlabeled time series data images. First, initialize the target detection model with a small set of labeled time series data images L, set the number of planned query samples n, and the variance threshold δ, and initialize the number of selected samples q to 0. Then, the target detection model is initialized using the labeled time series data image set L, and the prediction results for the image frames in the unlabeled time series data image set U are output. Next, according to the prediction result, the model uncertainty size of each iteration is calculated for each image frame in the unlabeled time series data image set U, and arranged in descending order. Take the sample with the largest model uncertainty. If the time series va...
PUM
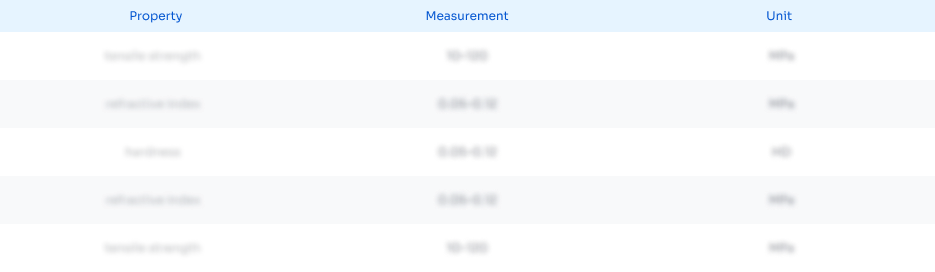
Abstract
Description
Claims
Application Information
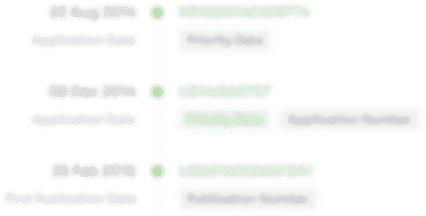
- R&D Engineer
- R&D Manager
- IP Professional
- Industry Leading Data Capabilities
- Powerful AI technology
- Patent DNA Extraction
Browse by: Latest US Patents, China's latest patents, Technical Efficacy Thesaurus, Application Domain, Technology Topic, Popular Technical Reports.
© 2024 PatSnap. All rights reserved.Legal|Privacy policy|Modern Slavery Act Transparency Statement|Sitemap|About US| Contact US: help@patsnap.com