Feature selection algorithm for particle swarm hybrid optimization in combination with collaborative learning strategy
A feature selection and particle swarm technology, applied in the field of machine learning, can solve the problems of high computing cost and low classification performance, achieve the effect of reducing classification cost, improving classification performance, and overcoming the tendency to fall into local optimal solutions
- Summary
- Abstract
- Description
- Claims
- Application Information
AI Technical Summary
Problems solved by technology
Method used
Image
Examples
Embodiment Construction
[0036] In order to make the purpose, technical solutions and advantages of the present invention clearer, the present invention will be further described in detail below in conjunction with the embodiments. Obviously, the described embodiments are only some embodiments of the present invention, rather than all embodiments. Based on the embodiments of the present invention, all other embodiments obtained by persons of ordinary skill in the art without making creative efforts belong to the protection scope of the present invention.
[0037] The feature selection algorithm (HPSO-CL) of particle swarm hybrid optimization combined with collaborative learning strategy includes the following steps:
[0038] Step 1: Prepare the dataset, using the colon dataset as an example, specifically:
[0039] The colon dataset is used to train and evaluate the performance of the proposed algorithm. The colon data set is a public data set obtained on Kaggle. The number of features of the data set...
PUM
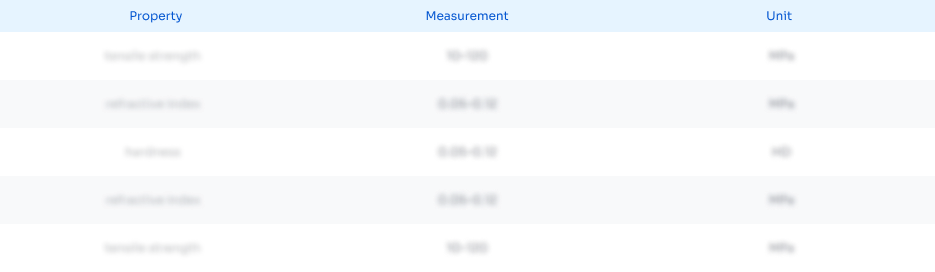
Abstract
Description
Claims
Application Information
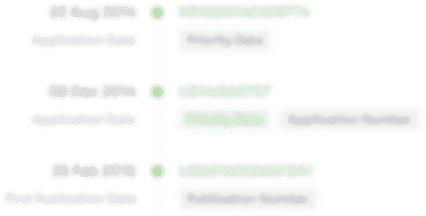
- R&D Engineer
- R&D Manager
- IP Professional
- Industry Leading Data Capabilities
- Powerful AI technology
- Patent DNA Extraction
Browse by: Latest US Patents, China's latest patents, Technical Efficacy Thesaurus, Application Domain, Technology Topic, Popular Technical Reports.
© 2024 PatSnap. All rights reserved.Legal|Privacy policy|Modern Slavery Act Transparency Statement|Sitemap|About US| Contact US: help@patsnap.com