Urban inland inundation rapid forecasting method based on multi-output machine learning algorithm
An urban waterlogging and machine learning technology, applied in the field of waterlogging simulation and urban waterlogging disaster prevention and control, can solve the problems of accuracy limitation, neglect of spatial correlation, complex algorithm model construction process, etc., to achieve fast and accurate forecasting, forecasting accuracy and timeliness Sex-enhancing effect
- Summary
- Abstract
- Description
- Claims
- Application Information
AI Technical Summary
Problems solved by technology
Method used
Image
Examples
Embodiment 1
[0049] A rapid prediction method of urban waterlogging based on multi-output machine learning algorithm, such as figure 1 shown, including the following steps:
[0050] S1. According to the data of the existing study area, construct an urban waterlogging model;
[0051] Collect the elevation, pipe network and land use data of the existing study area, use ArcGIS software to cut and extract the elevation and land use data, and correct the pipe network and check the topology.
[0052] The constructed urban waterlogging model is a one-dimensional hydrology-hydrodynamic coupling model based on SWMM and WCA2D; in this embodiment, as figure 2 As shown, taking a flood-prone area in Guangzhou as an example (with an area of 1.6 km 2 ), to construct a coupled model of urban waterlogging between one-dimensional pipe network SWMM and two-dimensional hydrodynamic WCA2D;
[0053] In the constructed urban waterlogging model, a storm runoff management model (SWMM) is constructed based on...
Embodiment 2
[0088] A rapid prediction method of urban waterlogging based on multi-output machine learning algorithm, such as figure 1 shown, including the following steps:
[0089] S1. According to the data of the existing study area, construct an urban waterlogging model;
[0090] The elevation, pipe network and land use data of the existing study area are collected and processed by ArcGIS software; the constructed urban waterlogging model is a one-dimensional hydrology and hydrodynamic coupling model based on SWMM and WCA2D; in this example, as Image 6 As shown, taking a watershed in Guangzhou as an example (with an area of 74 km 2 ), to build the urban one-dimensional pipeline network SWMM and the two-dimensional hydrodynamic WCA2D waterlogging coupling model; in the constructed urban waterlogging model, the storm runoff management model (SWMM) is constructed through the elevation, pipeline network and land use data; the rainfall data is input into The stormwater runoff management...
Embodiment 3
[0123] In this embodiment, the difference from Embodiment 1 is that in step S2, 1 year, 2 years, 5 years, 10 years, 20 years, 50 years, and 100 years (a total of 7) are used for different return periods, There are 56 rainfall scenarios in total.
[0124] To sum up, the simulation results of the multi-output random forest model and the urban waterlogging model have little difference and strong correlation. Under the full consideration of realistic scenarios, the prediction accuracy of spatial inundation water depth predicted by the multi-output random forest model is similar to that of the urban waterlogging model based on hydrology and hydrodynamics, but the computational efficiency of the former is much higher than that of the latter, and the prediction performance is better than that of the traditional method. Since the time required for the multi-output random forest model to simulate and predict the water depth is extremely short, and the accuracy also meets the requiremen...
PUM
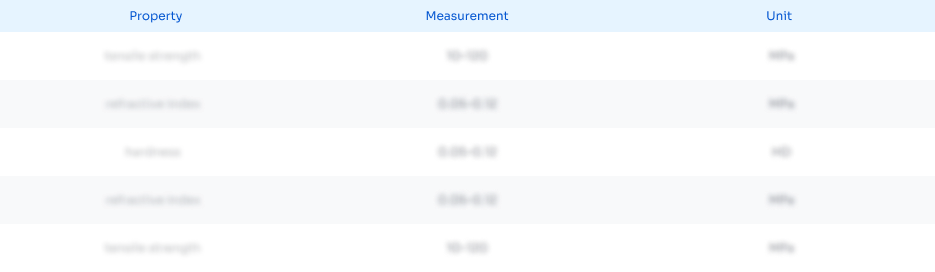
Abstract
Description
Claims
Application Information
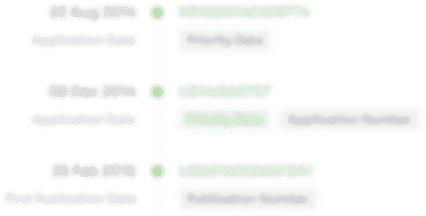
- R&D Engineer
- R&D Manager
- IP Professional
- Industry Leading Data Capabilities
- Powerful AI technology
- Patent DNA Extraction
Browse by: Latest US Patents, China's latest patents, Technical Efficacy Thesaurus, Application Domain, Technology Topic, Popular Technical Reports.
© 2024 PatSnap. All rights reserved.Legal|Privacy policy|Modern Slavery Act Transparency Statement|Sitemap|About US| Contact US: help@patsnap.com