Method for predicting time sequence of multiple measuring points on gas turbine based on steady-state characteristic composition
A steady-state feature, gas turbine technology, applied in forecasting, neural learning methods, neural architectures, etc., can solve problems such as not taking into account, and achieve the effect of global excellence, good generalization performance, and good prediction effect
- Summary
- Abstract
- Description
- Claims
- Application Information
AI Technical Summary
Problems solved by technology
Method used
Image
Examples
Embodiment Construction
[0021] The present invention will be further described below in conjunction with accompanying drawing and specific embodiment, but following embodiment does not limit the present invention in any way.
[0022] The design concept of the multi-measurement time series prediction method on the gas turbine based on steady-state feature composition proposed by the present invention is: extract the steady-state characteristics of the input samples in an end-to-end manner and construct an association relationship network, and guide the association relationship composition through the steady-state loss , establish a spatio-temporal neural network sequence prediction model based on the steady-state feature composition; use the spatio-temporal neural network sequence prediction model to extract the steady-state features of the system under constant working conditions, use spatio-temporal convolution for sequence prediction, and realize adaptive steady-state feature learning , and finally ...
PUM
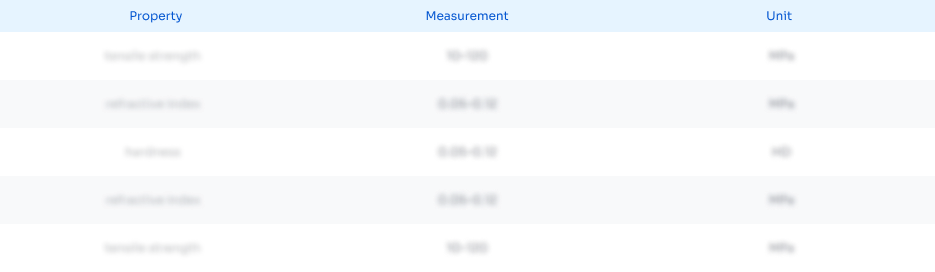
Abstract
Description
Claims
Application Information
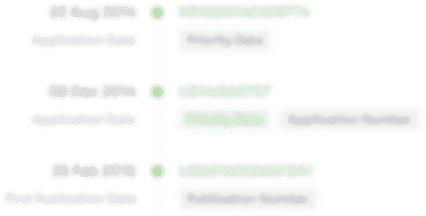
- R&D
- Intellectual Property
- Life Sciences
- Materials
- Tech Scout
- Unparalleled Data Quality
- Higher Quality Content
- 60% Fewer Hallucinations
Browse by: Latest US Patents, China's latest patents, Technical Efficacy Thesaurus, Application Domain, Technology Topic, Popular Technical Reports.
© 2025 PatSnap. All rights reserved.Legal|Privacy policy|Modern Slavery Act Transparency Statement|Sitemap|About US| Contact US: help@patsnap.com