Task-adaptive small sample image classification method based on meta transfer learning
A technology of transfer learning and classification method, applied in the field of task-adaptive small-sample image classification, it can solve the problems of insufficient extraction and unbalanced problems, and achieve the effect of good effect, fast learning and improved accuracy.
- Summary
- Abstract
- Description
- Claims
- Application Information
AI Technical Summary
Problems solved by technology
Method used
Image
Examples
Embodiment Construction
[0037] The present invention is further described below in conjunction with the accompanying drawings.
[0038] In order to solve the problem of insufficient feature extraction using shallow network extraction features by meta-learning method and the existing small sample learning method does not consider the imbalance problem in real scenarios, a task-adaptive small sample image classification method based on meta-transfer task-adaptive meta-learning ,MT-TAML) is proposed, and the technical solution adopted is as follows:
[0039] A task-adaptive small sample image classification method based on meta-transfer learning, the model population such as Figure 1 As shown, the model training process is as follows Figure 2 shown, including:
[0040] Step 1: Obtain a large-scale image dataset (such as ImageNet), pre-train the deep network using samples from the large-scale image dataset, and output a set of weight parameter vectors Θ and θ of feature extractors and classifiers;
[0041] ...
PUM
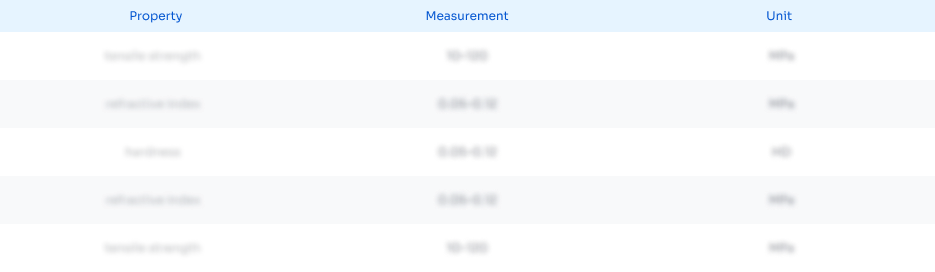
Abstract
Description
Claims
Application Information
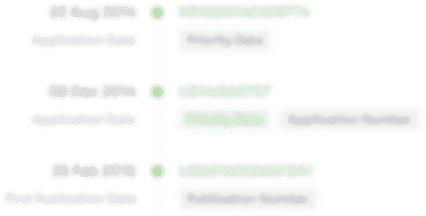
- R&D Engineer
- R&D Manager
- IP Professional
- Industry Leading Data Capabilities
- Powerful AI technology
- Patent DNA Extraction
Browse by: Latest US Patents, China's latest patents, Technical Efficacy Thesaurus, Application Domain, Technology Topic, Popular Technical Reports.
© 2024 PatSnap. All rights reserved.Legal|Privacy policy|Modern Slavery Act Transparency Statement|Sitemap|About US| Contact US: help@patsnap.com