Unbalanced load data type identification method based on VAE preprocessing and RP-2DCNN
A RP-2DCNN, load data technology, applied in 2D image generation, data processing applications, image data processing and other directions, can solve problems such as difficulty in extracting deep features, affecting load identification results, large running time, etc., to improve classification accuracy. rate, improve the effect of class imbalance problem
- Summary
- Abstract
- Description
- Claims
- Application Information
AI Technical Summary
Problems solved by technology
Method used
Image
Examples
Embodiment Construction
[0044] In order to make the technical means and effects realized by the present invention easy to understand, the present invention will be described in detail below in conjunction with the embodiments and accompanying drawings.
[0045]
[0046] figure 1 It is a flow chart of an unbalanced load data type identification method based on VAE preprocessing and RP-2DCNN in an embodiment of the present invention.
[0047] Such as figure 1 As shown, a method for identifying unbalanced load data types based on VAE preprocessing and RP-2DCNN in this embodiment includes the following steps: Step 1, collecting load data.
[0048] Step 2, preprocessing the load data.
[0049] Step 2 includes the following sub-steps:
[0050] Step 2-1, use the multi-order Lagrangian interpolation method to fill the missing values in the load data, the formula is as follows:
[0051]
[0052] Step 2-2, use the maximum and minimum values to normalize the load data to eliminate the impact of the...
PUM
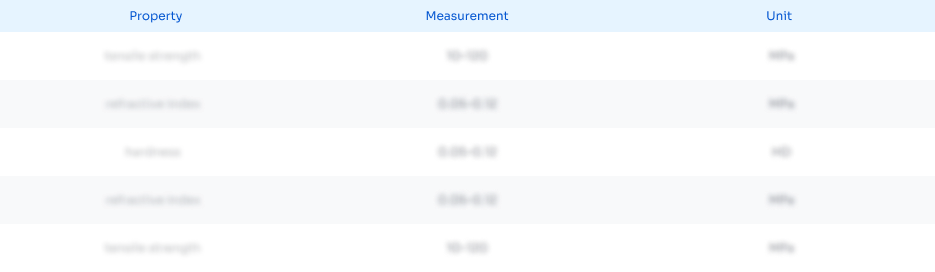
Abstract
Description
Claims
Application Information
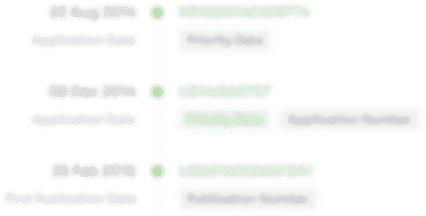
- R&D
- Intellectual Property
- Life Sciences
- Materials
- Tech Scout
- Unparalleled Data Quality
- Higher Quality Content
- 60% Fewer Hallucinations
Browse by: Latest US Patents, China's latest patents, Technical Efficacy Thesaurus, Application Domain, Technology Topic, Popular Technical Reports.
© 2025 PatSnap. All rights reserved.Legal|Privacy policy|Modern Slavery Act Transparency Statement|Sitemap|About US| Contact US: help@patsnap.com