Image denoising method and system
An image and image input technology, applied in the field of image processing, can solve the problems of long model training time, large memory consumption of deep convolutional neural networks, and difficulty in image denoising efficiency, so as to reduce parameters, improve effects, and reduce training parameters. Effect
- Summary
- Abstract
- Description
- Claims
- Application Information
AI Technical Summary
Problems solved by technology
Method used
Image
Examples
Embodiment 1
[0041] This embodiment provides an image denoising method, such as image 3 shown, including the following steps:
[0042] Step 1. Obtain an image data set, add noise, and obtain an image training set.
[0043] Specifically, additive white Gaussian noise is selected as the noise type for training, and noise is added to the clean images of the image dataset, and the training set is composed of the original image and the noisy image.
[0044] Step 2: Use the image training set to train the denoising model, and use the original image in the training set and the training sample pair of the noisy image as the input of the network structure. like figure 1 As shown, the network structure adopts the multi-scale feature extraction block (multi-scale feature extraction module) and the Ghost feature extraction block (Ghost feature extraction module) in turn. After performing multi-feature extraction and Ghost-based feature extraction on the noisy image, the extracted Noise features, t...
Embodiment 2
[0064] This embodiment provides an image denoising system, which specifically includes the following modules:
[0065] an image acquisition module configured to: acquire a noise image;
[0066] a denoising module, which is configured to: input the noise image into the denoising model to obtain the denoising image;
[0067] Among them, the denoising model passes through the multi-scale feature extraction block and the Ghost feature extraction block in turn, and after the feature extraction of the noise image, the noise feature is obtained, and the noise feature is subtracted from the noise image to obtain the denoised image.
[0068] It should be noted here that each module in this embodiment corresponds to each step in Embodiment 1 one by one, and the specific implementation process thereof is the same, which is not repeated here.
Embodiment 3
[0070] This embodiment provides a computer-readable storage medium on which a computer program is stored, and when the program is executed by a processor, implements the steps in the image denoising method described in the first embodiment above.
PUM
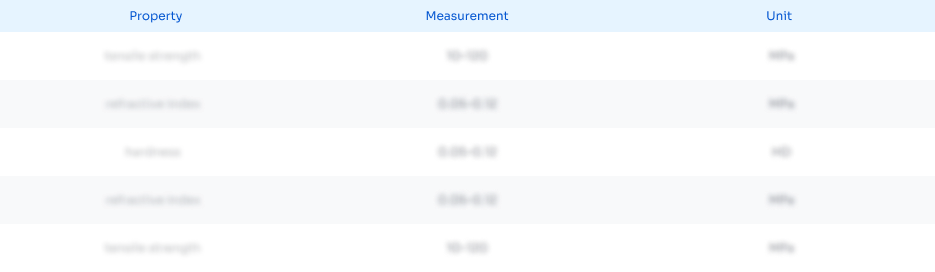
Abstract
Description
Claims
Application Information
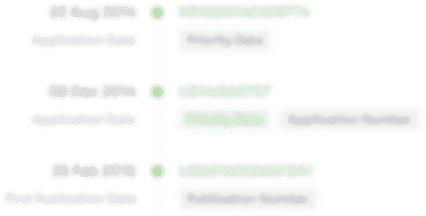
- R&D Engineer
- R&D Manager
- IP Professional
- Industry Leading Data Capabilities
- Powerful AI technology
- Patent DNA Extraction
Browse by: Latest US Patents, China's latest patents, Technical Efficacy Thesaurus, Application Domain, Technology Topic, Popular Technical Reports.
© 2024 PatSnap. All rights reserved.Legal|Privacy policy|Modern Slavery Act Transparency Statement|Sitemap|About US| Contact US: help@patsnap.com