Image recognition method of deep pulse neural network based on dynamic threshold neurons
A technology of spiking neural network and dynamic threshold, applied in the field of brain-like computing and deep learning, can solve the problems of low pulse rate, loss of conversion accuracy, low pulse transmission rate, etc., to achieve the effect of fast information transmission and improved accuracy
- Summary
- Abstract
- Description
- Claims
- Application Information
AI Technical Summary
Problems solved by technology
Method used
Image
Examples
Embodiment Construction
[0039] The present invention will be described in detail below with reference to the accompanying drawings and embodiments. At the same time, the technical problems and beneficial effects solved by the technical solution of the present invention are also described. It should be pointed out that the described embodiments are only intended to facilitate the understanding of the present invention, and do not have any limiting effect on it.
[0040] A dynamic threshold neuron model, as attached figure 1 As shown, the neuron model integrates the input pulses at each moment, and sets the threshold at this moment according to the change of the neuron membrane potential compared with the previous moment, and the change is equal to the input to the neuron at this moment. The pulse weighted sum, the threshold value is inversely proportional to the amount of change, when the membrane potential at this moment exceeds the calculated threshold value, a pulse is emitted, and the membrane pot...
PUM
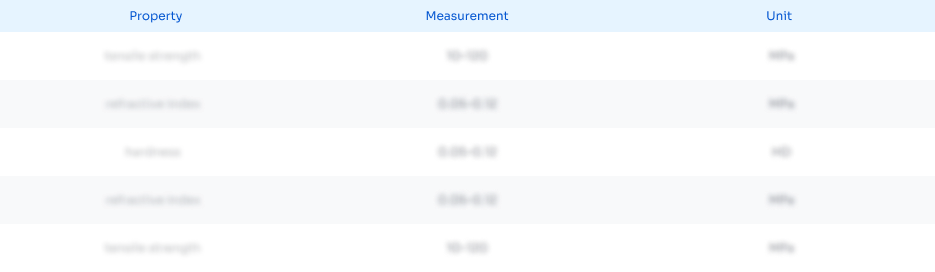
Abstract
Description
Claims
Application Information
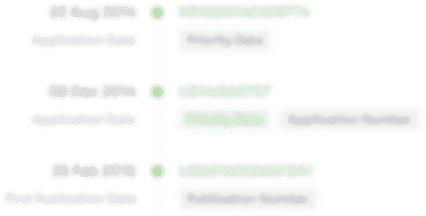
- R&D Engineer
- R&D Manager
- IP Professional
- Industry Leading Data Capabilities
- Powerful AI technology
- Patent DNA Extraction
Browse by: Latest US Patents, China's latest patents, Technical Efficacy Thesaurus, Application Domain, Technology Topic.
© 2024 PatSnap. All rights reserved.Legal|Privacy policy|Modern Slavery Act Transparency Statement|Sitemap