Self-supervised learning fine-grained image classification method based on twin network
A technology of supervised learning and twin networks, applied in the field of self-supervised learning fine-grained image classification based on twin networks, can solve problems such as difficult network loss functions, inability to accurately locate semantic components, and focus on subtle regional identification features to achieve improved classification Effects on Accuracy, Enhanced Robustness, and Generalization
- Summary
- Abstract
- Description
- Claims
- Application Information
AI Technical Summary
Problems solved by technology
Method used
Image
Examples
Embodiment 1
[0024] The self-supervised learning fine-grained image classification method based on the Siamese network according to the present invention comprises the following steps:
[0025] Step S1: Build an attention encoder, first extract the feature F of the input image by using the backbone network pre-trained on the large-scale classification dataset; the backbone network has a wide range of options, and you can choose the residual network (ResNet) and the classical depth Learning model VGG16 et al. The backbone network is pretrained on large-scale classification datasets such as ImageNet or Microsoft COCO. Second, the rich image information is captured by the bilinear pooling module. First, the image feature F is processed by a 1×1 convolutional layer (Conv), activation function (ReLU) and batch normalization (BN), thereby reducing the channel number, discard redundant information, and obtain attention map A with rich semantic information. Then, the image feature F and the atte...
Embodiment 2
[0052] Embodiment 2 of the present invention provides an electronic device, which is a memory and a processor, and is characterized in that, when a fine-grained image classification program based on self-supervised learning based on a twin network is stored and executed by the processor, the processor executes the algorithm based on the twin network. A self-supervised learning method for fine-grained image classification, which includes the following steps:
[0053] 1) Extract the feature representation of the input image using the constructed attention encoder;
[0054] 2) Obtain two different perspective samples of the image based on the attention and semantic erasure mechanism;
[0055] 3) Through the Siamese network, extract the sample features of different perspectives and guide the network to learn the perspective invariant features in a self-supervised manner;
[0056] 4) The central loss function is used to constrain the sample feature distance within the class;
[0...
Embodiment 3
[0059] Embodiment 3 of the present invention provides a computer-readable storage medium, characterized in that, when the program is executed by a processor, the processor is caused to execute a self-supervised learning fine-grained image classification method based on a twin network, and the method includes the following steps:
[0060] 1) Extract the feature representation of the input image using the constructed attention encoder;
[0061] 2) Using the attention mechanism and the semantic erasure mechanism to obtain two different perspective samples of the image;
[0062] 3) Through the Siamese network, extract the sample features of different perspectives and guide the network to learn the perspective invariant features in a self-supervised manner;
[0063] 4) The central loss function is used to constrain the sample feature distance within the class;
[0064] 5) The samples learned by the network and their features from different perspectives are sent to the classifier t...
PUM
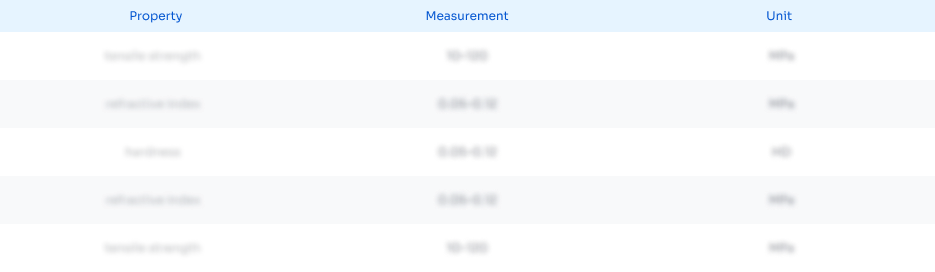
Abstract
Description
Claims
Application Information
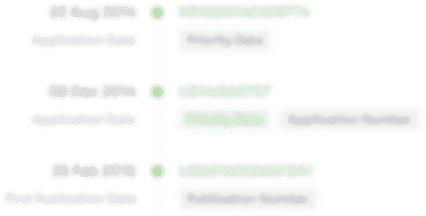
- R&D
- Intellectual Property
- Life Sciences
- Materials
- Tech Scout
- Unparalleled Data Quality
- Higher Quality Content
- 60% Fewer Hallucinations
Browse by: Latest US Patents, China's latest patents, Technical Efficacy Thesaurus, Application Domain, Technology Topic, Popular Technical Reports.
© 2025 PatSnap. All rights reserved.Legal|Privacy policy|Modern Slavery Act Transparency Statement|Sitemap|About US| Contact US: help@patsnap.com