Double-flow face counterfeiting detection method based on Swin Transform
A technology of forgery detection and dual-stream face, applied in neural learning methods, instruments, biological neural network models, etc., can solve problems such as insufficient generalization ability, poor anti-compression ability, weak generalization ability, etc., to improve generalization ability , resist compression, and enhance the effect of feature information
- Summary
- Abstract
- Description
- Claims
- Application Information
AI Technical Summary
Problems solved by technology
Method used
Image
Examples
Embodiment 1
[0033] see figure 1 , a dual-stream face forgery detection method based on Swin Transformer, including the following steps:
[0034] (1) Prepare the face video dataset:
[0035] Prepare the face forgery video dataset, and select the public face forgery video dataset: FaceForensics++;
[0036] (2) Crop the video into frames:
[0037] Considering that the training goal is to judge the authenticity of face images, the video data set is processed, specifically:
[0038] For all real or fake face videos, use the OpenCV tool library to divide them into non-overlapping video frames, and the specific method is to intercept at least 15 frames per second;
[0039] (3) Extract the face area:
[0040] Use the face detector to locate the face area, remove the surrounding irrelevant background information, extract the face area, and obtain the face area image dataset;
[0041] (4) Division of the dataset:
[0042] Use the face region image data set obtained in the previous step for tr...
Embodiment 2
[0049] This embodiment is basically the same as the first embodiment, and the special features are:
[0050] A dual-stream face forgery detection method based on Swin Transformer, comprising the following steps:
[0051] (1) Prepare the face video dataset:
[0052] First, we need to prepare the face forgery video dataset. The public face forgery video dataset is selected: FaceForensics++; the FaceForensics++ dataset is the first large-scale face forgery video dataset, which uses DeepFakes, FaceSwap, Face2Face, NeuralTextures to create different categories of face forgery techniques The first two techniques are facial identity replacement, and the latter two are facial expression reconstruction; this dataset also compresses the real and fake videos with three parameters of C0, C23, and C40 in H.264 encoding. It is to better simulate the quality of face videos spread on the Internet, in order to be closer to the face videos used in real life;
[0053] (2) Crop the video into ...
Embodiment 3
[0065] This embodiment is basically the same as the above-mentioned embodiment, and the special features are:
[0066] In this embodiment, a dual-stream face forgery detection method based on Swin Transformer, such as figure 1 shown, including the following steps:
[0067] First, the video data set needs to be preprocessed. The video data set here uses FaceForensics++. This data set contains four sub-data sets, namely DeepFakes and FaceSwap based on face swap forgery, and Face2Face based on facial expression reconstruction. And NeuralTexture, each video sub-dataset contains 1000 videos, plus 1000 real face videos, there are 5000 videos in total, which is a relatively large public video dataset. In order to simulate the video quality of real life scene propagation, this FaceForensics++ dataset also provides videos with three different compression qualities, namely C0 (no compression), C23 (light compression) and C40 (heavy compression).
[0068] The steps of video preprocessi...
PUM
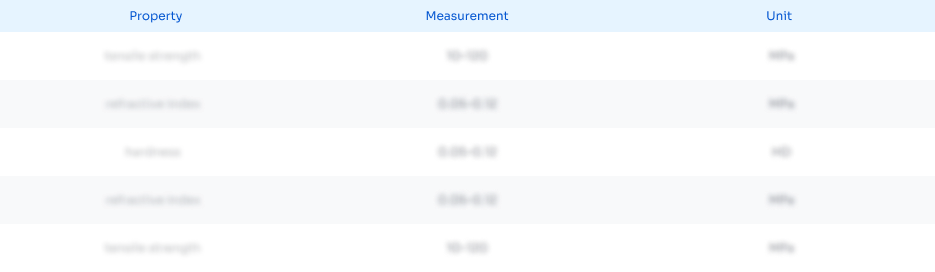
Abstract
Description
Claims
Application Information
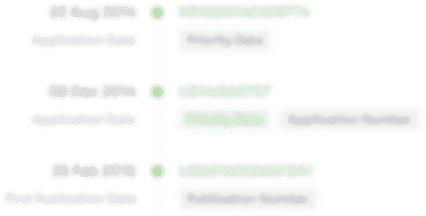
- R&D Engineer
- R&D Manager
- IP Professional
- Industry Leading Data Capabilities
- Powerful AI technology
- Patent DNA Extraction
Browse by: Latest US Patents, China's latest patents, Technical Efficacy Thesaurus, Application Domain, Technology Topic, Popular Technical Reports.
© 2024 PatSnap. All rights reserved.Legal|Privacy policy|Modern Slavery Act Transparency Statement|Sitemap|About US| Contact US: help@patsnap.com