Commodity recommendation method based on meta-learning and knowledge graph
A knowledge map and product recommendation technology, applied in the field of natural language processing, can solve problems such as limited number of products, sparse data, and reduced recommendation accuracy, so as to improve the overall effect, improve the quality of modeling, and improve the expressiveness
- Summary
- Abstract
- Description
- Claims
- Application Information
AI Technical Summary
Problems solved by technology
Method used
Image
Examples
Embodiment Construction
[0047] In this embodiment, a product recommendation method based on meta-learning and knowledge graph is to project users, products and related attributes of products into a latent semantic vector space with the same dimension, and then pass the transformer network and graph attention network to the The method of feature propagation and weighted combination aggregates the mutually influential feature vectors, so as to obtain the vector representation of user-item pairs with richer semantic information, which makes the recommendation system more accurate. Specifically, as figure 1 shown, proceed as follows:
[0048] Step 1: Obtain the data of the user's commodity historical interaction records. Each interaction record is composed of users and commodities. The users and commodities in the data are regarded as user nodes and commodity nodes respectively, and the historical interaction records in the data are regarded as user nodes and commodity nodes. An edge between them is rec...
PUM
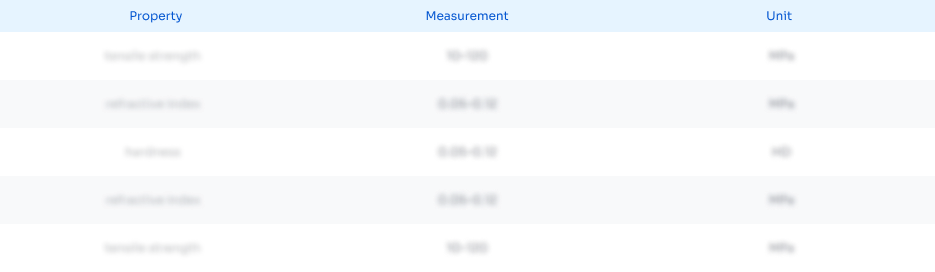
Abstract
Description
Claims
Application Information
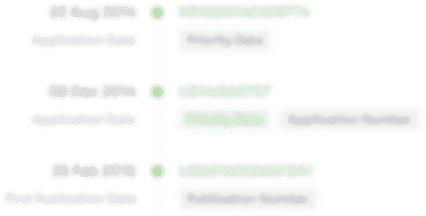
- R&D Engineer
- R&D Manager
- IP Professional
- Industry Leading Data Capabilities
- Powerful AI technology
- Patent DNA Extraction
Browse by: Latest US Patents, China's latest patents, Technical Efficacy Thesaurus, Application Domain, Technology Topic, Popular Technical Reports.
© 2024 PatSnap. All rights reserved.Legal|Privacy policy|Modern Slavery Act Transparency Statement|Sitemap|About US| Contact US: help@patsnap.com