Unsupervised image denoising method based on uncertainty perception
An uncertain and unsupervised technology, applied in the field of image denoising, can solve the problems of poor visual effect, no consideration of accidental uncertainty denoising network, etc., and achieve the effect of reducing interference
- Summary
- Abstract
- Description
- Claims
- Application Information
AI Technical Summary
Problems solved by technology
Method used
Image
Examples
Embodiment Construction
[0031] The embodiments of the present invention will be described in detail below, but the protection scope of the present invention is not limited to the examples.
[0032] The specific steps are:
[0033] (1) Before training, several smooth noise blocks with a size of 96×96 are extracted through the smooth noise block extraction rule (the number of noise blocks is closely related to the noise intensity). The original noisy image is randomly cropped into noise image blocks with a size of 96×96, and the number is 20,000, which is used as a training data set. The network model parameters are initialized by default.
[0034] (2) During training, randomly crop the 96×96 noise image and smooth the noise block to 64×64. The training period is 30, the initial learning rate is set to 0.001, and the decay rate is 0.5, which is decayed every ten epochs. Minimize the loss function using mini-batch stochastic gradient descent. The batch size is set to 128. The model adopts a staged ...
PUM
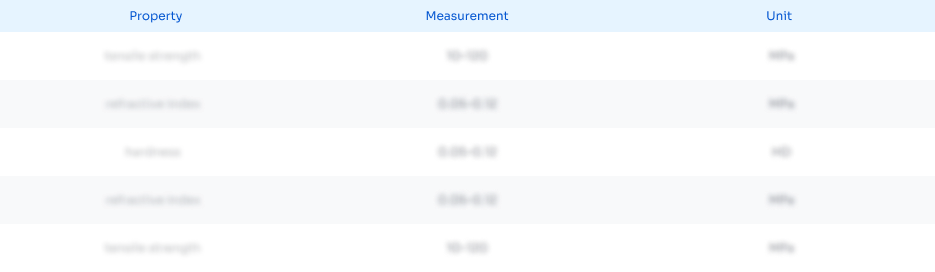
Abstract
Description
Claims
Application Information
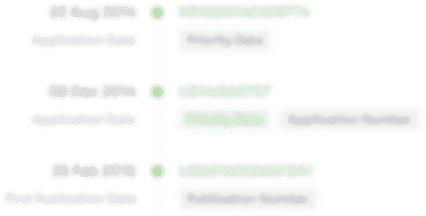
- R&D Engineer
- R&D Manager
- IP Professional
- Industry Leading Data Capabilities
- Powerful AI technology
- Patent DNA Extraction
Browse by: Latest US Patents, China's latest patents, Technical Efficacy Thesaurus, Application Domain, Technology Topic, Popular Technical Reports.
© 2024 PatSnap. All rights reserved.Legal|Privacy policy|Modern Slavery Act Transparency Statement|Sitemap|About US| Contact US: help@patsnap.com